In this section
- Disclaimer
- Analysis using administrative data
- Things you need to know about this release
- What data are currently available?
- The Generalised Structure Preserving Estimator (GSPREE)
- What the outputs show
- Conclusions and next steps
- Feedback
- Annex A: Further details on the Generalised Structure Preserving Estimator (GSPREE) model
1. Disclaimer
These Research Outputs are not official statistics. Rather they are published as outputs from research into an Administrative Data Census approach. These outputs must not be reproduced without this disclaimer and warning note and should not be used for policy- or decision-making.
Back to table of contents2. Analysis using administrative data
Ethnicity data are a high priority for users of census statistics. However, administrative data on ethnicity aren't widely collected and access to such data within the Office for National Statistics (ONS) is currently limited. To meet this need within an Administrative Data Census we’re investigating combining administrative and survey data to produce robust outputs.
The ONS Administrative Data Census project is working to assess whether the government-stated ambition that “censuses after 2021 will be conducted using other sources of data” can be met. We’re aiming to produce population estimates, household estimates and population and housing characteristics using a combination of administrative and survey data. This is to meet demands for improved population statistics and as a possible alternative to the census.
This analysis builds on a previous publication we released in August 2017. In that research, we used the Generalised Structure Preserving Estimator (GSPREE) small area estimation method with survey and administrative data. From this, we produced modelled estimates of ethnic group by local authority for 2011. Using 2011 data in this initial investigation allowed us to assess the approach by comparing modelled results with the 2011 Census. In this analysis, we’re taking the approach forward, producing estimates for 2015 and adjusting the model using the 2015 Statistical Population Dataset V2.0. This analysis is currently limited to England due to data availability.
Our findings show that the modelled estimates produce similar results to the census for the larger ethnic groups of “White”, “Black” and “Asian”. However, due to data limitations, the quality of the estimates varied – particularly for the smaller ethnic groups of “Mixed”, “Chinese” and “Other”. This shows promise for the ability to produce estimates from an Administrative Data Census. Figures produced using GSPREE were similar to alternative population estimates by ethnic group produced from the Annual Population Survey (APS PEEG). The GSPREE figures are less influenced by small or zero counts of some ethnicities than the APS PEEG estimates.
Back to table of contents3. Things you need to know about this release
- These Research Outputs are not official statistics – they’re published to demonstrate the type of analysis possible using administrative data.
- The analysis is for England only and the datasets used are the Annual Population Survey (APS), English School Census (ESC), 2011 Census and 2015 Statistical Population Dataset (SPD) V2.0.
- The only estimate of ethnicity that currently has National Statistics status is the census, but these figures can become outdated the further you move from the census date; this article provides an estimate of ethnicity by local authorities in England for 2015.
- Estimates for Wales were not produced for this publication due to data limitations, however we intend on producing these in the future.
- Further information on alternative estimates of ethnicity produced by the ONS can be found in our Population estimates by ethnic group methodology article.
4. What data are currently available?
Administrative data are collected for operational purposes. Our initial research found that ethnicity information isn’t widely collected and the statistical quality for the purpose of producing estimates varies.
The data currently available to us are:
- the 2011 Census, which provides large-scale data on ethnicity down to small geographical areas, however it is outdated by 2015
- the English School Census (ESC), which collects high quality information on ethnicity of school age children annually, with less than 1% missing data
- the Annual Population Survey (APS), which is a large household survey and can provide data at local authority level
- the Statistical Population Dataset (SPD) V2.0, which is a combination of administrative data sources produced by ONS’s Administrative Data Census project (this provides population totals by local authority, but doesn’t currently include any information on ethnicity)
Data limitations
English School Census (ESC) data cover school-age children. However, it doesn’t include children at independent schools, children having home schooling or those being educated somewhere other than at school.
Annual Population Survey (APS) data are from a much smaller sample than the census (approximately 285,000 respondents). This means the number of people sampled within detailed geographical areas and population subgroups is comparatively limited. The UK has an uneven distribution of population by ethnic group. It has an overall majority white population, while there are also small clusters of non-white groups living within small geographic areas.
This uneven distribution can cause problems when estimating non-white groups from survey samples in some geographic areas. Typically, survey data are more representative at national or regional level, but comparatively weaker for small areas or for smaller ethnic groups.
Some of the factors that may impact the estimation of ethnicity from the APS as opposed to using traditional census estimates are that:
- APS data are collected across the period of a year, compared with a single reference date for the census
- the census relies on people completing a questionnaire themselves compared with an interviewer-led survey used for the APS, and the questions are presented differently
- the APS is collected at a household level, and a clustering of single ethnicities within households can influence the estimates
- APS data don’t include all communal establishments (some information on students in halls of residence is collected through their families’ responses and nursing homes are covered), but they are all represented within census data
Table 1 shows differences in the distribution of the population by ethnic group between the 2011 Census and APS. In particular, a larger proportion of people described themselves as “Other” ethnic group in the APS compared with the 2011 Census. Also, a smaller proportion described themselves as “Mixed” in the APS. This is likely to be a result of the household-based sampling strategy used in the APS and comparatively small samples of smaller ethnic groups.
Ethnic group | ||||||
---|---|---|---|---|---|---|
Data source | White | Mixed | Asian | Chinese | Black | Other |
2011 Census | 85.42% | 2.25% | 7.10% | 0.72% | 3.48% | 1.03% |
Sep 2010 to Oct 2011 APS | 86.44% | 1.44% | 6.55% | 0.51% | 3.33% | 1.74% |
Download this table Table 1: Ethnic group distributions for England, 2011
.xls .csvThe Administrative Data Census project produces the Statistical Population Dataset (SPD) as part of the Census Transformation Programme. We produce SPD V2.0 by linking person records on administrative datasets. This builds on a previous methodology we used to produce SPD V1.0. The data used in SPD V2.0 include the Customer Information System, the Patient Register, English and Welsh School Census and Higher Education Statistics Agency datasets. However, the data linkage and estimation methods are still being developed and as such estimates produced are not official statistics. The SPD doesn’t currently include any information on ethnicity, but does provide population totals at local authority level and lower.
There are known quality issues with the SPD, for example the over estimate of working age males when compared with other official estimates. For SPD production, London is a particularly complex area due to the high level of migration in and out of the area. This isn’t always picked up in the administrative data sources in a timely manner. This results in an under-estimate in some London local authorities, particularly among men.
Coverage and data collection differences between the SPD, APS and census are likely to influence the results of this analysis.
Back to table of contents5. The Generalised Structure Preserving Estimator (GSPREE)
The Generalised Structure Preserving Estimator (GSPREE) is a small area estimation technique. We’re using this technique to combine data from multiple sources to produce estimates of ethnicity at local authority level. The GSPREE technique uses small area estimation to draw strength across multiple data sources, producing more robust estimates than may otherwise be possible. Previous releases described the use of the GSPREE method to produce ethnicity estimates for 2011 and 2014.
Current research
The current GSPREE research has produced estimates of ethnicity at local authority level for 2015. The year was chosen in line with data availability and to show the potential of the method to produce an estimate of ethnicity between censuses.
The model uses a combination of currently-available data sources. These are:
- the January 2015 English School Census (ESC)
- January to December 2015 Annual Population Survey (APS)
- 2011 Census
- 2015 SPD V2.0
Estimates are produced by modelling separately for three age groups: 0 to 4 years, 5 to 15 years and 16 and over. We chose these age groups to assess the strength of the model using different data sources. The model of those aged 5 to 15 benefits most from the ESC data. There’s comparatively less data available for those aged 0 to 4 years and 16 and over. We constructed the two models as shown in Figure 2.
Model Inputs | |||
---|---|---|---|
Model | Year | Inputs | Benchmark Data |
1 | 2015 | 2015 APS 2015 ESC | 2015 APS - ethnic group 2015 SPDV2.0 - population by local authority |
2 | 2015 | 2015 APS 2015 ESC 2011 Census | 2015 APS - ethnic group 2015 SPDV2.0 - population by local authority |
Download this table Table 2: Model 1 and model 2 data inputs, 2015
.xls .csvYou can find further information on the structure, application and performance of the Generalised Structure Preserving Estimator (GSPREE) models in Annex A. Overall our findings show that for model 1, the base APS data needed the least adjustment by the model for those aged 5 and 15. This is because there were strong ESC data available for these ages. Conversely, the most adjustment needed in model 1 was for those aged 16 and over as the data available for these ages were weaker. Model 2 had the extra census data available, which reduced the amount of adjustment needed for all age groups.
Confidence intervals for the Generalised Structure Preserving Estimator (GSPREE) estimates
Confidence intervals are used to express the statistical accuracy of an estimate. If an estimate has a high level of error, the corresponding 95% confidence interval will be very wide. A “bootstrapping” method was used to estimate them for the Generalised Structure Preserving Estimator (GSPREE) estimates, which involves resampling. You can find further details on this method in the previous methodological publication. Figures 1 and 2 show the confidence intervals around the GSPREE estimates for models 1 and 2 by ethnic group.
Figure 1: GSPREE Model 1 (APS and ESC) estimates with 95% confidence intervals, 2015
England
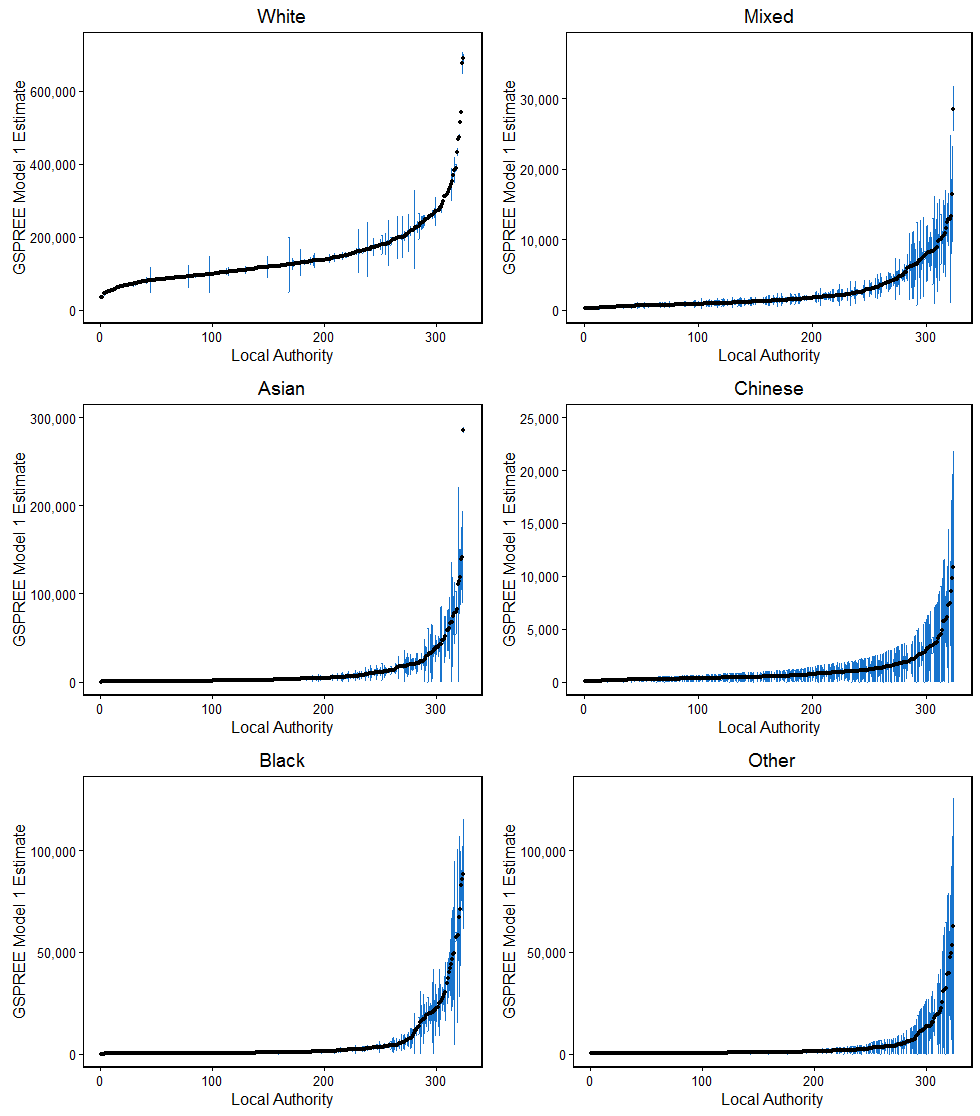
Source: Office for National Statistics
Notes:
- GSPREE – Generalised Structure Preserving Estimator.
- APS – Annual Population Survey.
- ESC – English School Census.
- There are different y axis scales across the 6 plots.
- The local authorities are ranked by the size of the estimate.
- Confidence interval data are available to download.
Download this image Figure 1: GSPREE Model 1 (APS and ESC) estimates with 95% confidence intervals, 2015
.png (21.3 kB)
Figure 2: GSPREE Model 2 (APS, ESC and 2011 Census) estimates with 95% confidence intervals, 2015
England
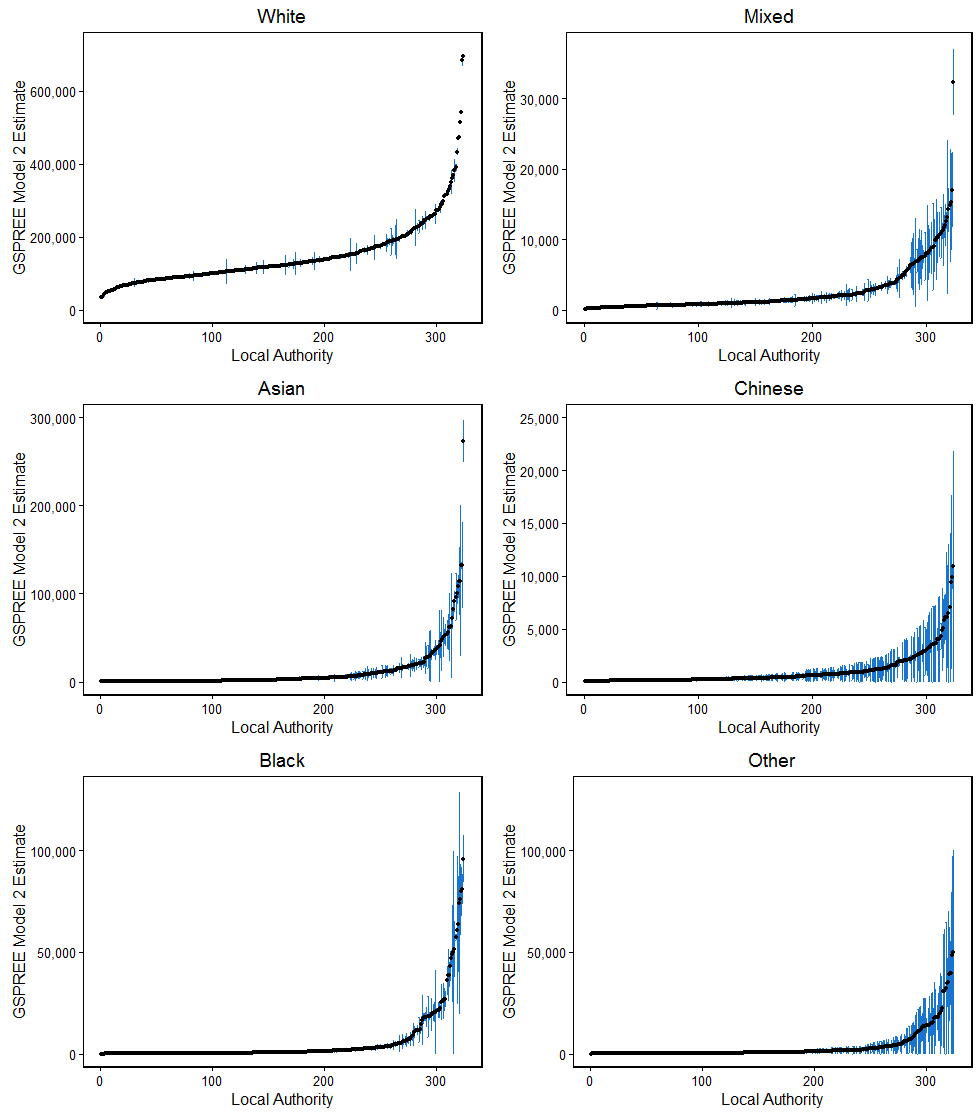
Source: Office for National Statistics
Notes:
- GSPREE – Generalised Structure Preserving Estimator.
- APS – Annual Population Survey.
- ESC – English School Census.
- There are different y axis scales across the 6 plots.
- The local authorities are ranked by the size of the estimate.
- Confidence interval data are available to download.
Download this image Figure 2: GSPREE Model 2 (APS, ESC and 2011 Census) estimates with 95% confidence intervals, 2015
.png (20.9 kB)Overall, the confidence intervals around the model 1 GSPREE estimates are wider without the inclusion of the census data as a proxy source. This means they have larger errors. This can be clearly seen with the “White” ethnic group estimates. The confidence intervals around some local authorities, specifically those with smaller proportions of “White” population, are wider with model 1.
The confidence intervals for “Chinese” and “Other” are very wide for both models. The survey data contain very small sample counts for “Chinese”, and there are also many local authorities where there are no “Chinese” respondents in the survey. The “Other” ethnic group is difficult to estimate as it differs between data sources. The differences in the mode of collection of the data source and the ethnic group question may influence the estimation of this ethnic group.
The local authorities with large estimates of “Black” population also have very wide confidence intervals. This occurred as the majority of local authorities have very small “Black” population estimates, and a small number have very large population estimates. The two extremes in population size were more distinct compared with the other ethnic groups, and this is difficult to capture.
The quality of the estimate can also be assessed using the coefficient of variation (CV). This is the ratio of the standard error of an estimate to the estimate itself, expressed as a percentage. A smaller CV indicates a higher-quality estimate. The table of GSPREE estimates for model 1 had 50.6% of estimates with CVs less than 20%. Model 2 had 64.8% of estimates less than 20%. For both models, most of the high CVs were for the estimates of the “Chinese” and “Other” ethnic groups.
Back to table of contents6. What the outputs show
The results in Figure 3 show how the modelled estimates compare between models 1 and 2. In the diagrams, each point represents a local authority. The dashed blue diagonal line of Y equals X shows perfect agreement between the two models. Where the yellow line of best fit is the same as the Y equals X line, a perfect match has been made. If, however, the yellow line falls below the Y equals X line, model 1 is producing a lower estimate than model 2. Conversely, when the line is above Y equals X, model 1 is producing a higher estimate than model 2.
As the graphs show, the model predicts relatively consistently between models 1 and 2. This suggests that despite the strength of the census data in model 2, model 1 is capable of producing very similar results without using these data. This is particularly the case for the larger ethnic groups of “White”, “Asian” and “Black” where the results from the two models are consistent. The results for “Mixed”, “Chinese” and “Other” were found to vary the most between the two models, as there is a larger spread around the line of best fit. This could be the result of a comparatively small proportion of the population identifying as (in particular) “Mixed” and “Chinese”. Additionally, this may suggest a difference in how people select their ethnicity responses between the census and APS datasets.
Figure 3: GSPREE model 1 and 2 estimates comparison, 2015
England
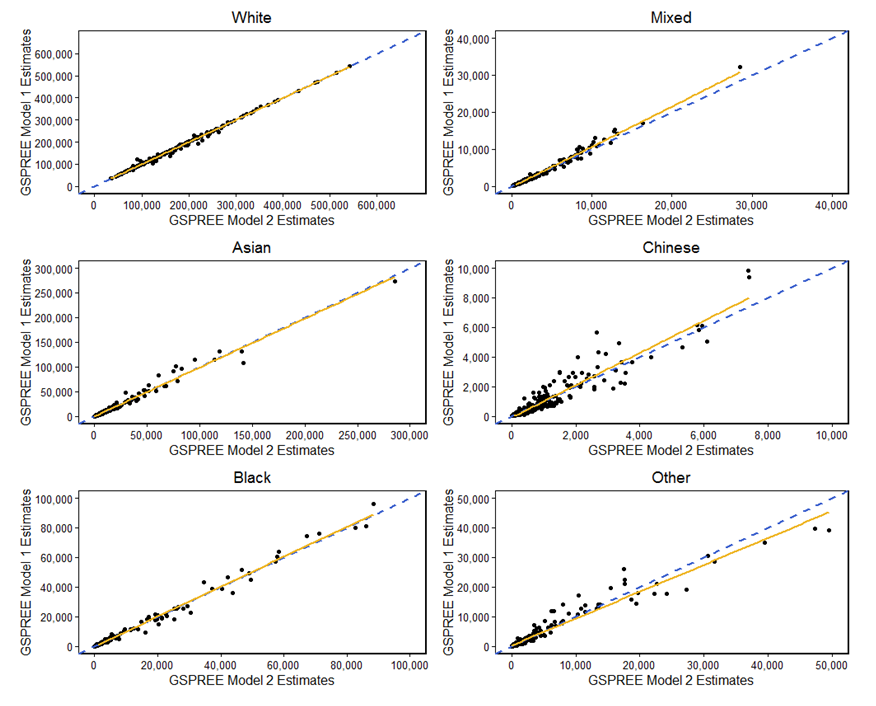
Source: Office for National Statistics
Notes:
- GSPREE – Generalised Structure Preserving Estimator.
Download this image Figure 3: GSPREE model 1 and 2 estimates comparison, 2015
.png (176.9 kB)Comparison with alternative ethnicity estimates
Figures 4 and 5 compare the ethnicity estimates we produced using the Generalised Structure Preserving Estimator (GSPREE) method with alternative population estimates by ethnic group. The comparison estimates were constructed from the Annual Population Survey (APS PEEG) and 2011 Census, and are referred to here as the APS PEEG method. Where the yellow line falls below the Y equals X line, the GSPREE estimate is lower than the estimate produced by the APS PEEG method. Alternatively, when the line is above Y equals X, the GSPREE estimate is higher than the estimate produced by the APS PEEG method. The GSPREE and APS PEEG methods estimated for slightly different ethnic groups, so the comparisons in the figures below are with the five common ethnic groups.
It’s worth noting that comparisons between the two models can only be made where figures are available for both models. The APS PEEG estimates are based on the APS which suffers from low or zero sample counts for smaller ethnic groups in a large number of local authorities. This means that a large number of APS PEEG estimates weren’t available for this comparison.
As shown in Figures 4 and 5, the results produced using the APS PEEG and GSPREE methods are largely consistent. This is especially the case for the larger ethnicities of “White”, “Asian” and “Black”. While the average estimate is relatively consistent between the two methods for those identifying as “Other” ethnic group, the spread of estimates is comparatively wide. This could be due to the volatility of estimates in this comparatively small group. In particular, the APS PEEG estimates are primarily based on the APS which is a household-level survey, leading to clustering of ethnicities within sampled households.
Results between GSPREE models 1 and 2 and the APS PEEG were fairly similar. Most notably, model 1 produced a slightly lower estimate for the “Mixed” ethnicity than model 2. The strength of the correlation of the points was also weaker in the model 1 comparison.
Figure 4: GSPREE model 1 versus APS PEEG estimates, 2015
England
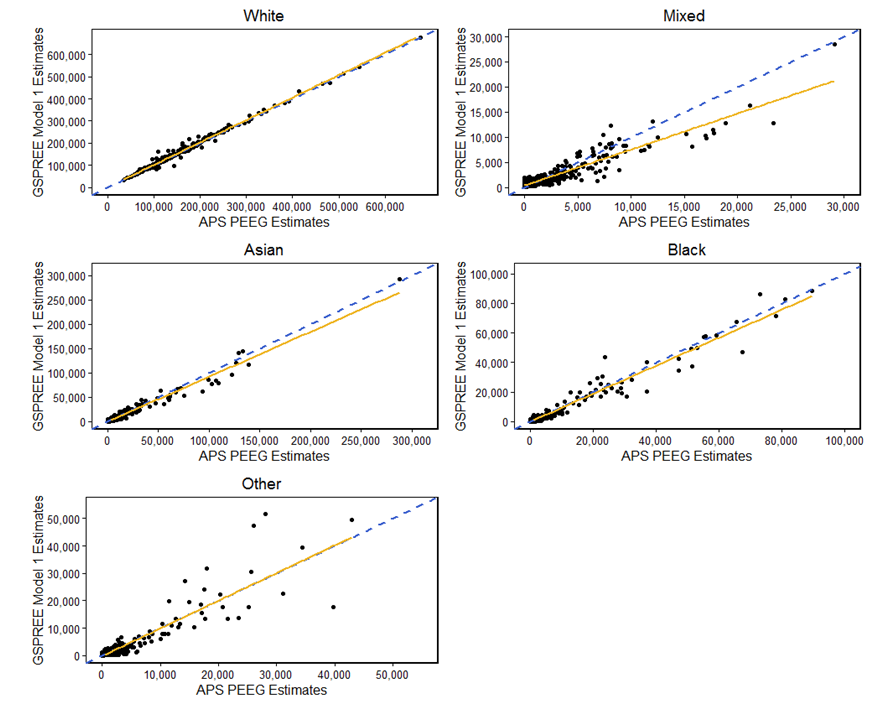
Source: Office for National Statistics
Notes:
- GSPREE – Generalised Structure Preserving Estimator.
- APS PEEG – Annual Population Survey based population estimates by ethnic group estimates.
- To allow comparison with APS PEEG estimates, the GSPREE estimates of “Asian” and “Chinese” ethnic groups have been combined into the “Asian” group.
Download this image Figure 4: GSPREE model 1 versus APS PEEG estimates, 2015
.png (153.8 kB)
Figure 5: GSPREE model 2 versus APS PEEG estimates, 2015
England
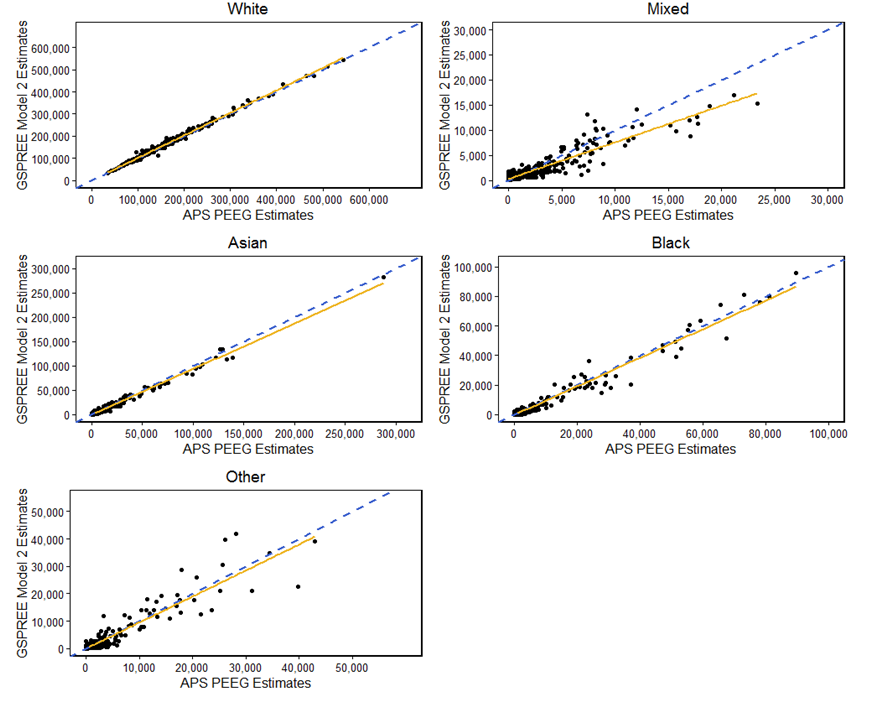
Source: Office for National Statistics
Notes:
- GSPREE – Generalised Structure Preserving Estimator.
- APS PEEG – Annual Population Survey based population estimates by ethnic group estimates.
- To allow comparison with APS PEEG estimates, the GSPREE estimates of “Asian” and “Chinese” ethnic groups have been combined into the “Asian” group.
Download this image Figure 5: GSPREE model 2 versus APS PEEG estimates, 2015
.png (152.8 kB)Figure 6 shows case studies of ethnicity estimates for 2015 against the 2011 Census for four local authorities in England. These cover Waverley, Birmingham, Manchester and Kensington and Chelsea.
The graph for Waverley shows a predominantly white population, with all models estimating over 95% of the population being “White”. In the context of a large “White” population, the GSPREE models 1 and 2 and APS PEEG results are similar. Interestingly for Waverley, the APS PEEG estimates have a substantially higher “Other” population than the GSPREE models and the 2011 Census. APS PEEG estimate 2,900 “Other” individuals, compared with 300 for GSPREE model 1, 600 for GSPREE model 2 and 400 for the 2011 Census.
While we may expect the figures to have increased slightly since the 2011 Census due to population growth, this proportional growth is very large. However, we know that the September 2010 to October 2011 APS over counted “Other” compared with the 2011 Census (Table 1). Additionally, as no contact was made with any “Black” individuals in Waverley in the APS, the APS PEEG estimate for this area is 0.
The population of Birmingham shows a markedly different trend. Both the GSPREE and APS PEEG models estimate that there has been an increase in the proportion of “White” residents of Birmingham since the 2011 Census. This may be due to the “White” category used being quite broad and therefore encompassing a diverse group of people. Also, there may be differences in classification in the other sources used by the models compared with the classification used in the 2011 Census.
Conversely, the models compared here may be estimating a true decline in the proportion of, in particular, “Asian” and “Mixed” people in Birmingham. However, we know the 2010 to 2011 APS undercounted “Mixed” compared with the 2011 Census (Table 1).
Estimates from APS PEEG for Manchester show a notably larger “Asian” population compared with the GSPREE results. The APS PEEG figures estimate 99,300 “Asian” people, compared with 85,800 in GSPREE model 1 and 81,900 in GSPREE model 2. The GSPREE models indicate a larger proportion in the “Other” population when compared with the 2011 Census and the results generated by APS PEEG.
The results from Kensington and Chelsea are of particular importance for the GSPREE model. As the GSPREE models here are adjusted with the Statistical Population Dataset (SPD), some weaknesses of the SPD are carried forward. A good example of this is areas of London with high levels of migration. There are also specific local authority level effects such as a low count that has been identified in Kensington and Chelsea.
As the SPD is created from administrative data sources such as tax records and registration with NHS services, some people aren’t picked up by the SPD. They are, therefore, under counted by the GSPREE model. This lower population is most apparent in GSPREE model 1. GSPREE model 2 is less affected by this lower population as the model includes 2011 Census data in the modelling procedure as a robust data source. However, the figures are adjusted with the SPD after the modelling procedure. This means the final result of both models 1 and 2 were lower than the census and APS PEEG estimates.
Figure 6: Ethnicity proportion estimates versus 2011 Census, 2015
England
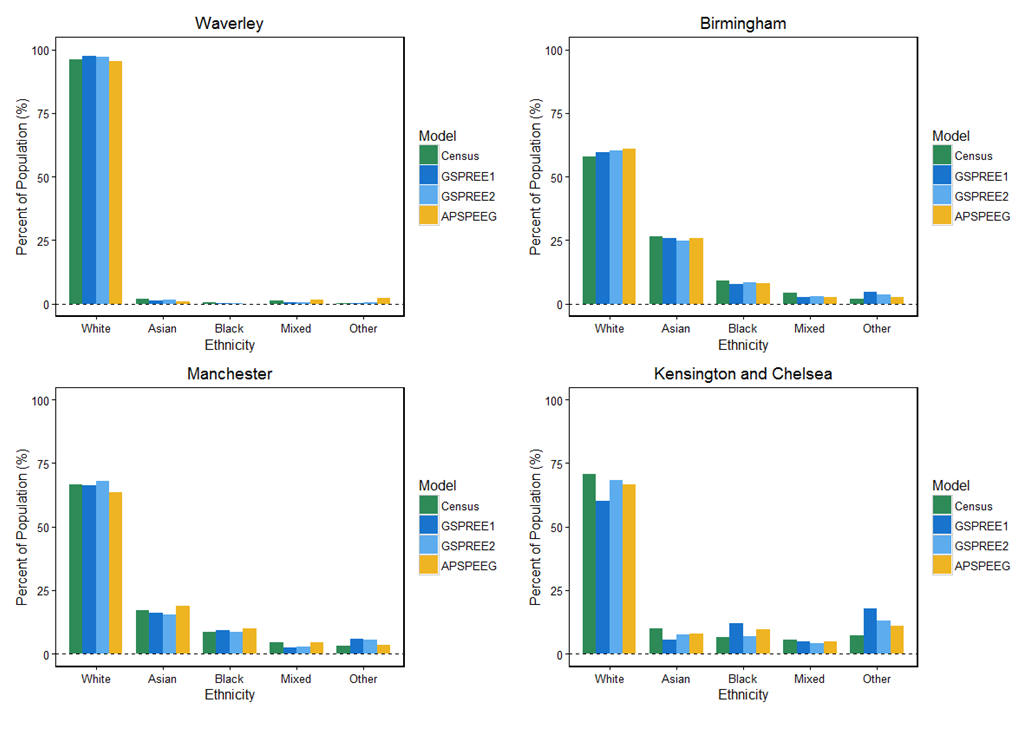
Source: Office for National Statistics
Notes:
- Census – 2011 Census estimates.
- GSPREE1 – Generalised Structure Preserving Estimator model 1 estimates.
- GSPREE2 – Generalised Structure Preserving Estimator model 2 estimates.
- APSPEEG – Annual Population Survey based population estimates by ethnic group estimates.
- To allow comparison with APS PEEG estimates, the GSPREE estimates of “Asian” and “Chinese” ethnic groups have been combined into the “Asian” group.
Download this image Figure 6: Ethnicity proportion estimates versus 2011 Census, 2015
.png (116.2 kB)7. Conclusions and next steps
Using administrative and survey data with the Generalised Structure Preserving Estimator (GSPREE) method shows promise for the ability to produce mid-decade estimates of ethnicity by local authority. In particular, the model didn’t generate substantially different results between including and not including the census data. This suggests the method may be suitable for producing estimates of ethnicity from an Administrative Data Census. However, without the census the confidence intervals around the estimates were found to be slightly wider.
As the estimates produced by GSPREE are based on statistical modelling, it’s possible to produce robust confidence intervals for the data. This is more challenging for estimates produced from survey or administrative data alone as there’s a number of factors that may influence confidence in the results. This may include differences in a wide range of factors such as the context of how questions are asked or the populations sampled within a survey.
However, the survey data are less accurate for smaller ethnicities due to small sample sizes, which means the model estimates are also less reliable. We should continue to encourage improved collection of and access to ethnicity data, such as hospital episode statistics where ethnicity is already collected. In particular, we should seek to improve data covering smaller and harder to reach groups.
We’re considering a number of next steps:
- producing figures for Wales using the Welsh School Census, APS, 2011 Census and the SPD
- simulating a population characteristic survey from the 2011 Census in place of the APS data; this reflects a long term goal of the Administrative Data Census project to use a population characteristic survey alongside the SPD to collect data that would be difficult to get from alternative sources
- investigating the use of a more flexible version of GSPREE to relax some of the assumptions
- rolling forward census attributes; for characteristics that could be assumed to be stable over time such as country of birth or ethnicity, we may be able to carry forward data from a previous census to the current SPD population
We’d appreciate your feedback on these and other approaches.
Back to table of contents8. Feedback
We’re keen to get your feedback on these Research Outputs and the methodology used to produce them. This includes how they might be improved and potential uses of the data. Please email your feedback to Admin.Data.Census.Project@ons.gov.uk. Please include the title of the output in your response.
Back to table of contents9. Annex A: Further details on the Generalised Structure Preserving Estimator (GSPREE) model
Model 1 uses the January to December 2015 Annual Population Survey (APS) and January 2015 English School Census (ESC). Model 2 includes the same sources as model 1, but also includes the 2011 Census as a robust but outdated data source. By including the potentially outdated census in model 2, we were able to investigate the effect of including the robust but outdated data source and whether the distributions defined by the model were significantly changed. This allowed us to test the operation and stability of the model and assess the effect of including census data on the results.
The data structure used by the GSPREE models to update the tables of ethnicity by local authority is shown in Figure 7. The estimation begins in step one with survey data from the target year (in this case, 2015). This provides up-to-date estimates that are representative of the population, but lacks robust and complete detail at a small area level.
At step two, the relationship between the survey data and the association structure of the proxy data source(s) is used to obtain the best estimates of the target cross-tabulated distributions. This makes the best use of all available data sources. The association structure is the transformation of the cross tabulation shown in the proxy data source(s), where the row and column (local authority and ethnic group) information is taken into account.
The proxy data sources provide granular detail, but don’t cover the whole population (for example, the ESC only provides ethnicity for children), or are for a different time period. From the model relationship between the survey data and the association structure, the modelled estimates are compiled in step three. Finally at step four, these estimates are adjusted with robust and recent row (2015 SPD) and column (2015 APS) population estimates to produce final estimates.
Figure 7: Current GSPREE data structure for models 1 and 2, 2015
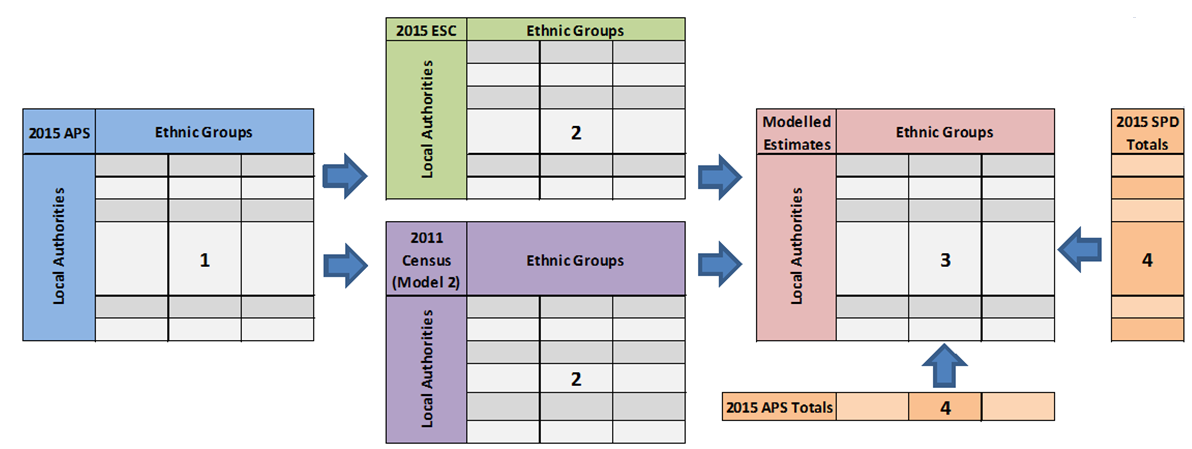
Source: Office for National Statistics
Notes:
- APS – Annual Population Survey.
- ESC – English School Census.
- SPD – Statistical Population Dataset v2.0.
Download this image Figure 7: Current GSPREE data structure for models 1 and 2, 2015
.png (94.2 kB)Figure 10 shows the results for models 1 and 2, detailing the adjustment of the association structure based on the APS information. The association structure is the relationship between the base data (2015 APS) and the proxy data source(s) (2015 ESC and in model 2 the 2011 Census). An adjustment value of 1 indicates the association structure didn’t need any adjustment based on the APS information. However, the further this value is from 1, the more adjustment was needed compared with the other age groups in the model. You can find further detail on this in a previous methodological publication.
The 5 to 15 age group required the smallest adjustment to derive the result in model 1. This was likely due to the influence of the ESC as the most robust data source available for this age group. The ESC is used for every age group on the model, without a strong data source available in the 16 and over population. However, the degree of adjustment to derive the result was greater.
Overall however, model adjustment was significantly smaller in model 2 than in model 1. This was due to the relative strength and proximity of the census data in the estimation. With the 2011 Census in model 2, the 16 and older age group needed very little adjustment.
Figure 8: Adjustment of association structure in models 1 and 2, 2015
England
Source: Office for National Statistics
Notes:
- Model adjustment represents the adjustment of the association structure, based on APS data.
- A model adjustment value of one indicates no adjustment.
- The further the model adjustment value varies from one, the greater the adjustment.
- APS – Annual Population Survey.
Download this chart Figure 8: Adjustment of association structure in models 1 and 2, 2015
Image .csv .xlsThe impact of including the census is backed up by the findings in Figure 9, showing how much model 2 relied on the supporting data (2015 ESC and 2011 Census). Weight values closer to 1 represent heavy reliance on that data source. As can be seen from the graph, all age groups in model 2 were heavily reliant on the census data, as a robust data source. The use of an outdated census is likely to be more influential in 2015 than was found in the previous publication for 2011 which used the 2001 Census. This is likely to be due to the 2011 Census being significantly closer to the 2015 modelled date. The reliance on the 2011 Census was particularly notable for those aged 16 and over. This is again likely to be due to the lack of other data to accurately characterise this population.
Figure 9: Reliance of model 2 on supporting data, 2015
England
Source: Office for National Statistics
Notes:
- The weight represents the data reliance of the model on the supporting data.