Table of contents
- Disclaimer
- Main points
- Things you need to know about this release
- The Data for Children Partnership
- What is included in the Proof of Concept (PoC)
- Feasibility analysis of the Proof of Concept (PoC) dataset
- Quality and methodology
- Linkage results
- Lessons learned and next steps
- Annex A: Glossary
- Annex B: Highest educational attainment of child by Local Authority 2011
1. Disclaimer
These Research Outputs are not official statistics. Rather they are published as outputs from a proof of concept feasibility study exploring the use of administrative data linked to the 2011 Census. These outputs should not be used for policymaking or decision-making. This work uses research datasets that may not exactly reproduce National Statistics aggregates.
It is important that the research presented here be read alongside the quality and methodology information in Section 7 to help interpretation and avoid misunderstanding. These outputs must not be used without this disclaimer and warning note.
This work contains statistical data from the Office for National Statistics (ONS), which is Crown Copyright. The use of the ONS statistical data in this work does not imply the endorsement of the ONS in relation to the interpretation or analysis of the statistical data. This work uses research datasets that may not exactly reproduce National Statistics aggregates.
Back to table of contents2. Main points
There is currently a need for research to better understand the barriers and gateways to social mobility to inform public policy targeted at disadvantaged children and young people.
This report contains outcomes of analysis and descriptions of the linkage methodology used.
This Proof of Concept (PoC) dataset brings together information relating to personal characteristics of children and their family members with their educational attainment; this enables insights to better understand the effect of factors such as personal and familial characteristics, and geography on educational outcomes.
The analysis of the PoC datasets was conducted on personal characteristics, educational attainment, household characteristics, vulnerable groups and geography.
Deterministic linking was used to match the 2011 Census and a bespoke extract of the feasibility AEDE to form the Growing Up in England (GUIE) dataset; a high linkage rate of 90% was achieved.
Prior to linkage, a series of preparatory steps were taken; these are described in further detail in Section 7: Quality and methodology.
Any limitations and issues identified will be considered in future iterations of the GUIE dataset.
3. Things you need to know about this release
In our role as the largest producer of independent official statistics in the UK, the Office for National Statistics (ONS) provides the data and analysis that help us understand how people in the UK experience life. This information has traditionally come from surveys or the census, however, these sources often cannot provide enough detail or timeliness. For this reason, we are developing our use of administrative and linked data, and this article presents research into how census-linked educational datasets might be used in the future.
The Growing Up in England (GUIE) dataset was produced in partnership with Administrative Data Research UK (ADR UK) as part of the Data for Children Partnership. The data were accessed for research purposes through the ONS’s Secure Research Service (SRS).
This analysis has been produced in collaboration with the ONS Centre for Equalities and Inclusion. The aim of this centre is to work with other researchers to ensure that the right data are available to address the main social and policy questions about fairness and equity in society.
These research outputs are not official statistics, however these new data have the potential to give us far better insight into some of the factors affecting educational attainment. It is important to understand that the Proof of Concept (PoC) dataset created from this innovative linkage project into educational attainment and progression has some limitations and issues. Any disparities between groups in the level and progression of attainment presented in this research may be because of other characteristics rather than the one being directly measured and compared. The outputs are published to demonstrate the type of analysis possible using administrative data and the ADR UK investment. As such, these results should not be used to draw conclusions about educational outcomes of children and their corresponding characteristics, and instead are illustrative of the population sizes we expect to capture within the data.
Back to table of contents4. The Data for Children Partnership
The Data for Children Partnership is a strategic partnership between Administrative Data Research UK (ADR UK), the Office of the Children’s Commissioner for England (CCO) and other parties such as academics, charities and government departments. The objectives of the partnership are to:
ensure policy that concerns children and young people in England is informed by high-quality, relevant data
unblock barriers to data sharing through ensuring relevant data collection and raising the profile of the importance of sharing and linking data from across the government
deliver impact through data from contributing to intelligence-led policy and achieving demonstrable outcomes from research
ADR UK is a partnership transforming the way researchers access the UK’s wealth of public sector data to enable better informed policy decisions that improve people’s lives; it is formed by three national partnerships (ADR Scotland, ADR Wales, and ADR NI), and the ONS. Ultimately, ADR UK is creating a sustainable body of knowledge about how our society and economy function by linking together data held by different parts of the government, and by facilitating safe and secure access for accredited researchers to these newly joined-up data sets. These are tailored to decision makers’ needs, to provide answers required to solve policy questions.
We provide a range of services for ADR UK, specifically:
We acquire permission from data suppliers for their administrative or survey records to be used for research purposes; the legal gateway for suppliers to provide access to their data through the ONS is the Statistics and Registration Service Act (SRSA) 2007 as amended by the Digital Economy Act (DEA) 2017.
As a data processor we clean, link and de-identify the data according to specifications defined by the researchers prior to the data being made accessible; key words such as data linkage and de-identified are defined in Annex A.
The SRS gives accredited or approved researchers secure access to de-identified, unpublished data in order to work on research projects for the public good and provides a safe setting, as part of the Five Safes Framework to protect data confidentiality (the framework is a set of principles adopted by a range of secure labs, including the ONS; most datasets are available to access through secure remote access to the SRS and in some instances, the data can only be accessed from an approved safe setting)
The Office of the Children’s Commissioner for England (CCO) is responsible for speaking up for children and young people so that policymakers and the people who have an impact on their lives take their views and interests into account when making decisions about them. The CCO is developing a vulnerability database to shine a light on the extent and impact of child vulnerability in England.
The purpose of this report is to present the research outcomes of a feasibility study on a dataset that links a feasibility version of the All Education Dataset for England (AEDE) to the 2011 Census by describing the datasets used for linkage, analysis, linkage methodology, and next steps.
The Proof of Concept (PoC) analysis discussed in this research output was carried out under the third objective of the Data for Children Partnership in collaboration with the Centre for Equalities and Inclusion.
Back to table of contents5. What is included in the Proof of Concept (PoC)
For this Proof of Concept, data from the 2011 Census have been linked to education and attainment information from a bespoke extract of the feasibility All Education Dataset for England (AEDE) data from the Department for Education (DfE). Further information on the feasibility AEDE can be found on the published Feasibility AEDE source overview.
Linking the feasibility AEDE data to the Census brings personal, family and household characteristics together with educational attainment information. This is illustrated in Figure 1 , where the blue boxes highlight the data included in the dataset.
Figure 1: Data included in the GUIE PoC feasibility dataset
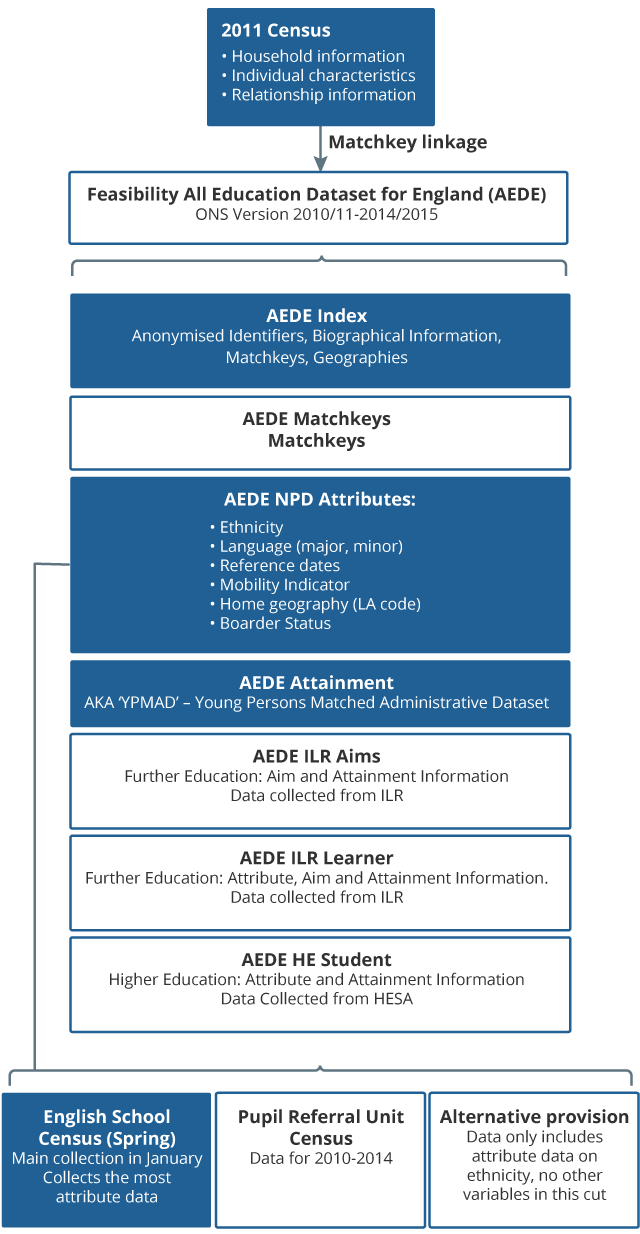
Source: Office for National Statistics
Notes:
- NPD is the National Pupil Database, ILR is Individualised Learner Record (Further Education data) and HE is Higher Education data.
- Boxes highlighted in blue indicate data included in the GUIE PoC dataset.
Download this image Figure 1: Data included in the GUIE PoC feasibility dataset
.png (77.6 kB)All Education Dataset for England (AEDE)
Created by the DfE, the feasibility AEDE is a large longitudinal record-level education dataset that covers government-funded education in England up to the academic year 2014/15. The dataset is created from the National Pupil Database (NPD), Further Education (FE) and Higher Education (HE) data.
The NPD is an administrative datastore that is held by the DfE and includes English school census and attainment information from the Young Person’s Matched Administrative Dataset (YPMAD); students’ socio-demographic characteristics are obtained from the termly school census, pupil referral unit and alternative provision censuses – these are linked to attainment data recorded by awarding bodies.
FE data comprise of Individualised Learner Record (ILR) and include socio-demographic characteristics of individuals in further education and work-based learning in England and attainment information.
HE data are collected by the Higher Education Statistics Agency (HESA) all government-funded higher education institutes in the UK are required to send data to HESA as well as further education institutes where higher education is delivered; HE data contain information on the socio-demographic characteristics of students and any qualifications obtained.
All personal identifiers in the feasibility AEDE held by the ONS are pseudonymised (made non-identifiable) to ensure confidentiality; the method used ensures identifiable information is not revealed but can be used for data linkage.
For the purposes of this PoC, only data from the NPD have been linked to the 2011 Census. Consequently, the feasibility AEDE extract for this PoC includes only spring English School Census (ESC), Key stage 4 (KS4) and Key stage 5 (KS5) attainment data from the NPD – FE and HE data are not included.
English School Census
The ESC is a collection of pupil- and school-level information. The PoC collection includes:
secondary
middle-deemed secondary
local authority maintained special and non-maintained special schools
academies including free schools
studio schools, university technical colleges and city technology colleges in England; service children’s education schools may also participate on a voluntary basis
The data are collated by Local Authorities (LAs) into electronic returns and submitted to the DfE via a secure online data transfer system. There is published information on School Census: Data quality and processing, which is collected each term (that is, three times a year), however the data used in PoC relate to the January (Spring term) data collection.
The ESC does not include data from independent schools, and only collects information about individuals attending state-funded schools in England. The information on these individuals includes:
To summarise, the ESC extract included in the PoC contains information only on ethnicity, language and mobility and it does not include information on FSM, SEN or exclusions.
Attainment data
Attainment data for KS4 and KS5 are submitted to the DfE from approximately 150 awarding bodies; the attainment data are then linked to student data.
For the purpose of this report, the data used for this linkage will be referred to as feasibility AEDE, although noting that it is not the full feasibility AEDE, but the bespoke extract described above.
2011 Census
The census takes place every 10 years and gives the most accurate estimate of all the people and households in England and Wales. It provides a snapshot of family make-up and relationships within households as well as demographic and socio-economic characteristics for almost the entire population.
The 2011 Census holds much valuable information including ethnicity, main language spoken, country of birth, and religion to name just a few. Although the 2011 Census data is nearly 10 years old, the information remains relevant as some personal characteristics (such as country of birth) are generally stable over time. The 2011 Census took place on 27 March 2011, and the population of England and Wales on this day was 56,075,912. There is published information on how ONS processed the information for the 2011 Census.
Proof of Concept (PoC) coverage
The analysis presented in this report focused on the feasibility of using this type of linked data to understand factors associated with educational attainment. The linked PoC dataset contains approximatively 2 million children in 2011 and 8 million household members. The size of the populations used in the analysis were slightly lower than these figures, because of issues identified post-linkage. The analysis section below provides more information on the sample sizes and issues identified.
The size of the longitudinal sample allows for multiple disaggregation which would not be possible using traditional survey data, with a more limited sample size.
Only pseudonymised data has been used in this analysis and results are shown at an aggregated level, so individuals cannot be identified.
Students are contained in the linked PoC dataset if they were present in the 2011 Census and enrolled in government-funded education in England at any point between the academic years 2010 /11 and 2014/15. For 2011, this creates a cohort of children enrolled in school aged between 13 and 18 years in KS4 or KS5. A representation of the age groups included in the PoC dataset is shown in Figure 2.
Further details on linkage methodology, results and quality can be found in Section 7: Quality and Methodology.
Figure 2: Age cohort contained in the GUIE PoC feasibility dataset at start of academic year
Office for National Statistics
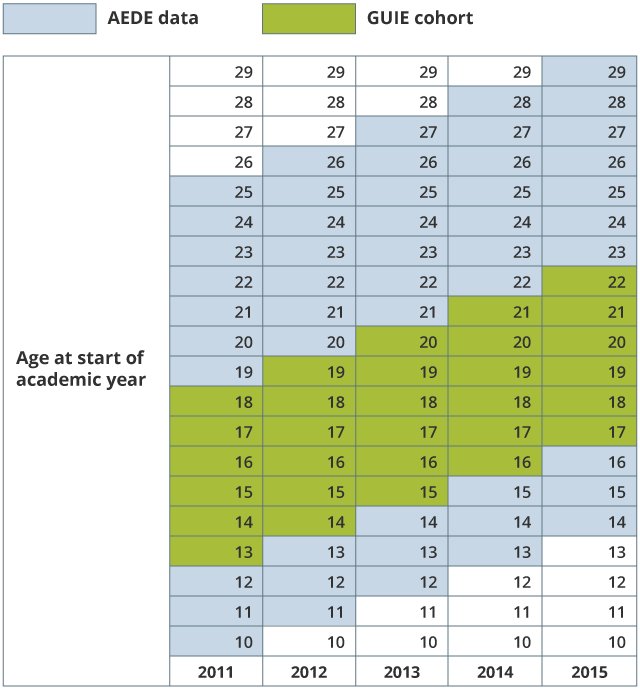
Source: Office for National Statistics
Download this image Figure 2: Age cohort contained in the GUIE PoC feasibility dataset at start of academic year
.png (34.4 kB)6. Feasibility analysis of the Proof of Concept (PoC) dataset
A child’s socioeconomic background is an important determinant of their chances of future life success. The performance gap between advantaged and disadvantaged children develops at an early age and widens throughout pupils’ lives. Research is therefore needed to better understand the barriers and gateways to social mobility to inform public policy targeted at disadvantaged children and young people.
Currently, there are a number of data gaps within the existing evidence base that have prevented research from being conducted into the interaction between characteristics, such as religion and family background, and educational attainment. Where data are available for important characteristics, often sample sizes prevent the ability of researchers to produce robust estimates for the smallest groups within our society, meaning that these groups are not routinely reflected in the statistics produced.
By bringing together information relating to the personal characteristics of children and their family members with their educational attainment, this dataset is uniquely placed to provide insight to better understand the effect of factors such as personal and familial characteristics, and geography on educational outcomes, increasing our understanding of the nuanced interactions between factors that lead to disadvantage throughout the life course. Early research to demonstrate the potential of administrative data to provide information on educational qualification, collected by the census since 1961 was published in October 2019.
The feasibility analysis of this Proof of Concept (PoC) dataset was carried out in collaboration with the Centre for Equalities and Inclusion. The aim of this analysis was to assess whether this new linked dataset added value to the existing evidence base surrounding the educational outcomes of children. To ensure this analysis reflected the requirements of researchers across the field, our analysis was steered by a working group of representatives from across government, academia and third sector organisations. We are grateful to the working group for their input over the course of the analysis.
Because of the limitations of the PoC dataset, the numbers provided in this section are solely illustrative and should only be used to provide researchers intending to conduct analysis on future iterations of the dataset with an estimate of the size of their populations of interest. The results in this section should not be used to make assumptions about the interaction between a child’s personal or familial characteristics and their associated educational attainment.
It is important to note that the characteristics of household members are as reported in Census 2011, while educational attainment information is taken from a longitudinal source and could have been attained in any of the academic years between 2010/11 and 2014/15. It is also possible that the characteristics recorded for children were assigned to them by a parent or other household member, rather than by the child self-identifying.
Throughout this section, numbers have been rounded to the nearest multiple of 10 as per the Secure Research Service’s (SRS’s) standard rules on Statistical Disclosure Control (SDC) to ensure information is not identifiable.
Measuring educational attainment
For this analysis, educational attainment was measured using the levels defined in Table 1.
Level | Description | Number of children |
---|---|---|
Below Level 1 | Equivalent to entry level qualifications or no qualifications | 20,830 |
Level 1 | One to four GCSEs (any grade) or equivalent | 127,380 |
Level 2 | Five or more GCSEs (grade A* to C) or equivalent (including intermediate apprenticeships) | 474,260 |
Level 3 | Two or more A-levels or equivalent | 1,040,700 |
Missing | No attainment identified | 79,620 |
Download this table Table 1: Educational attainment levels with descriptions and associated population counts within the PoC dataset
.xls .csvInitially the scope of the feasibility analysis included level of attainment by Key stage 4 (KS4), Key stage 5 (KS5), and a measure of progress between KS4 and KS5. However, as the attainment variables used are cumulative and do not have an associated key stage indicator, while it would have been possible to use age as a proxy for key stage, it would not have been possible to differentiate between when a qualification of the same level was attained. For example, if a child attained a “Level 3” qualification in KS4, there would be no way to identify whether this same child had attained another “Level 3” qualification in KS5.
In addition, the PoC also does not include attainment for all respondents at KS5, as some children will have completed their education in an educational setting that is not captured in the PoC, for example an independent school or a school outside of England, or may not have gone on to complete KS5.
Given the cumulative nature of the attainment data, it was not possible to tell whether a child had dropped out of the dataset, potentially having achieved higher level qualifications elsewhere, or just had not attained any higher-level qualifications at KS5. For these reasons, the analysis instead covers highest educational attainment within the period the individual is captured within the dataset.
Personal characteristics and educational attainment
Because of the way in which the data were linked, the PoC dataset is not considered representative for those aged 13 years as of 31 August 2011, and so this analysis covers only children aged 14 to 18 years as of 31 August 2011. A number of children also had to be excluded from the analysis because of issues that arose post-linkage, which meant it was not possible to combine their educational attainment information with their corresponding characteristics collected from the Census. This resulted in the total population of cohort children within the PoC dataset falling from approximately 1.9 million to approximately 1.7 million children.
Tables 2 to 10 provide information on the population sizes of children within the PoC dataset broken down by a range of personal characteristics and educational attainment.
Sex | Highest level of educational attainment | |||||
---|---|---|---|---|---|---|
Below Level 1 | Level 1 | Level 2 | Level 3 | Missing | Total | |
Male | 12,900 | 77,100 | 257,930 | 485,780 | 49,530 | 883,240 |
Female | 7,890 | 50,040 | 215,460 | 553,270 | 29,900 | 856,550 |
Invalid | 40 | 240 | 870 | 1,660 | 190 | 3,000 |
Download this table Table 2: Number of children by highest level of educational attainment and sex of child
.xls .csv
Religion | Highest level of educational attainment | |||||
---|---|---|---|---|---|---|
Below Level 1 | Level 1 | Level 2 | Level 3 | Missing | Total | |
No religion | 6,680 | 44,830 | 148,120 | 262,500 | 29,920 | 492,060 |
Christian | 10,320 | 64,290 | 258,280 | 590,270 | 37,960 | 961,120 |
Buddhist | 60 | 240 | 1,020 | 3,510 | 130 | 4,960 |
Hindu | 180 | 500 | 2,770 | 18,450 | 280 | 22,180 |
Jewish | 40 | 170 | 810 | 3,750 | 100 | 4,850 |
Muslim | 1,690 | 7,900 | 27,390 | 73,980 | 4,880 | 115,830 |
Sikh | 80 | 560 | 2,680 | 12,190 | 270 | 15,780 |
Other | 40 | 220 | 910 | 2,630 | 130 | 3,930 |
Not stated/missing | 1,760 | 8,680 | 32,290 | 73,420 | 5,940 | 122,090 |
Download this table Table 3: Number of children by highest level of educational attainment and religion of child
.xls .csv
Country of Birth | Highest level of educational attainment | |||||
---|---|---|---|---|---|---|
Below Level 1 | Level 1 | Level 2 | Level 3 | Missing | Total | |
England | 17,880 | 116,680 | 435,280 | 939,860 | 71,740 | 1,581,450 |
Northern Ireland | 30 | 110 | 380 | 810 | 60 | 1,380 |
Scotland | 130 | 450 | 1,610 | 4,140 | 310 | 6,630 |
Wales | 130 | 460 | 1,440 | 3,330 | 310 | 5,680 |
UK (otherwise not specified) | <10 | <10 | 30 | 80 | <10 | 110 |
Ireland | 20 | 80 | 270 | 640 | 70 | 1,070 |
Other EU: Member countries in March 2001 | 330 | 1,020 | 4,340 | 12,780 | 690 | 19,160 |
Other EU: Accession countries April 2001 to March 2011 | 420 | 1,450 | 4,880 | 9,220 | 1,230 | 17,200 |
Other countries | 1,280 | 4,390 | 17,480 | 55,360 | 3,120 | 81,630 |
Missing | 600 | 2,730 | 8,560 | 14,500 | 2,080 | 28,460 |
Download this table Table 4: Number of children by highest level of attainment and country of birth
.xls .csv
Main language | Highest level of educational attainment | |||||
---|---|---|---|---|---|---|
Below Level 1 | Level 1 | Level 2 | Level 3 | Missing | Total | |
English | 17,230 | 117,670 | 441,630 | 972,600 | 71,960 | 1,621,080 |
Welsh | <10 | <10 | <10 | <10 | <10 | 30 |
Other UK language | 20 | 10 | <10 | 20 | <10 | 60 |
French | 40 | 70 | 360 | 920 | 70 | 1,450 |
Portuguese | 70 | 310 | 860 | 1,320 | 210 | 2,770 |
Spanish | 30 | 70 | 300 | 760 | 70 | 1,220 |
Other European language (EU) | 450 | 1,310 | 4,550 | 8,610 | 1,190 | 16,120 |
Other European language (non EU) | 20 | 60 | 270 | 770 | 40 | 1,160 |
Other European language (non-national) | 120 | 450 | 1,430 | 3,110 | 360 | 5,480 |
West/Central Asian language | 80 | 370 | 1,270 | 2,600 | 390 | 4,710 |
South Asian language | 330 | 1,700 | 6,170 | 18,520 | 980 | 27,700 |
East Asian language | 60 | 180 | 820 | 2,540 | 130 | 3,740 |
Oceanic/Australian language | <10 | <10 | <10 | <10 | <10 | <10 |
North/South American language | <10 | <10 | <10 | <10 | <10 | 20 |
Caribbean Creole | <10 | <10 | <10 | <10 | <10 | <10 |
African language | 150 | 480 | 1,880 | 4,530 | 420 | 7,450 |
Other languages | 30 | 30 | 90 | 200 | 30 | 370 |
Sign language | 490 | 60 | 160 | 110 | 130 | 940 |
Missing | 1,730 | 4,620 | 14,450 | 24,060 | 3,620 | 48,480 |
Download this table Table 5: Number of children by highest level of attainment and main language of child
.xls .csv
English language proficiency | Highest level of educational attainment | |||||
---|---|---|---|---|---|---|
Below Level 1 | Level 1 | Level 2 | Level 3 | Missing | Total | |
Very well | 3,390 | 29,950 | 113,390 | 272,670 | 18,320 | 437,720 |
Well | 1,250 | 3,060 | 8,780 | 11,040 | 3,370 | 27,500 |
Not well | 1,330 | 290 | 650 | 440 | 870 | 3,580 |
Not at all | 1,550 | 30 | 80 | 40 | 90 | 1,780 |
Failed multiple ticking rules/missing | 13,310 | 94,050 | 351,360 | 756,510 | 56,970 | 1,272,210 |
Download this table Table 6: Number of children by highest level of attainment and English language proficiency of child
.xls .csv
Disability status | Highest level of educational attainment | |||||
---|---|---|---|---|---|---|
Below Level 1 | Level 1 | Level 2 | Level 3 | Missing | Total | |
Yes, limited a lot | 9,330 | 3,110 | 6,520 | 6,170 | 7,870 | 33,000 |
Yes, limited a little | 1,930 | 6,190 | 15,890 | 20,620 | 7,440 | 52,060 |
No | 8,490 | 112,430 | 434,020 | 983,620 | 60,060 | 1,598,630 |
Failed multiple ticking rules/ missing | 1,090 | 5,640 | 17,830 | 30,290 | 4,240 | 59,090 |
Download this table Table 7: Number of children by highest level of attainment by disability status of child
.xls .csv
Carer status | Highest level of educational attainment | |||||
---|---|---|---|---|---|---|
Below Level 1 | Level 1 | Level 2 | Level 3 | Missing | Total | |
No | 19,240 | 117,890 | 441,040 | 978,680 | 73,170 | 1,630,010 |
Yes, 1 to 19 hours a week | 230 | 3,330 | 13,420 | 28,720 | 1,830 | 47,530 |
Yes, 20 to 49 hours a week | 50 | 560 | 1,800 | 2,750 | 390 | 5,540 |
Yes, over 50 hours a week | 80 | 360 | 1,150 | 1,870 | 280 | 3,740 |
Failed multiple ticking rules/ missing | 1,230 | 5,250 | 16,860 | 28,680 | 3,950 | 55,970 |
Download this table Table 8: Number of children by highest level of attainment by carer status of child
.xls .csv
General health | Highest level of educational attainment | |||||
---|---|---|---|---|---|---|
Below Level 1 | Level 1 | Level 2 | Level 3 | Missing | Total | |
Very good | 7,250 | 86,570 | 343,470 | 822,230 | 45,170 | 1,304,680 |
Good | 6,180 | 32,160 | 106,720 | 184,700 | 23,670 | 353,430 |
Fair | 3,880 | 4,770 | 12,520 | 15,760 | 6,300 | 43,220 |
Bad | 1,540 | 920 | 2,210 | 2,290 | 1,820 | 8,780 |
Very bad | 1,290 | 220 | 500 | 550 | 530 | 3,080 |
Failed multiple ticking rules/ missing | 690 | 2,730 | 8,840 | 15,180 | 2,140 | 29,590 |
Download this table Table 9: Number of children by highest level of attainment by general health of child
.xls .csv
Ethnicity (Census) | Highest level of educational attainment | |||||
---|---|---|---|---|---|---|
Below Level 1 | Level 1 | Level 2 | Level 3 | Missing | Total | |
English/ Welsh/ Scottish/ Northern Irish/ British | 15,110 | 102,730 | 384,920 | 792,800 | 62,660 | 1,358,210 |
Irish | 50 | 240 | 950 | 3,930 | 150 | 5,320 |
Gypsy or Irish Traveller | 240 | 280 | 530 | 240 | 400 | 1,690 |
Any other White background | 20 | 70 | 250 | 600 | 60 | 1,000 |
White and Black Caribbean | 390 | 2,450 | 8,210 | 13,450 | 1,920 | 26,410 |
White and Black African | 110 | 480 | 1,740 | 4,510 | 380 | 7,200 |
White and Asian | 190 | 850 | 3,430 | 11,310 | 540 | 16,320 |
Any other Mixed / Multiple ethnic background | 20 | 90 | 250 | 480 | 60 | 900 |
Indian or British Indian | 320 | 1,240 | 6,170 | 33,110 | 620 | 41,460 |
Pakistani or British Pakistani | 700 | 3,480 | 11,830 | 30,800 | 2,020 | 48,830 |
Bangladeshi, British Bangladeshi | 230 | 1,450 | 4,790 | 14,280 | 740 | 21,480 |
Chinese | 50 | 100 | 700 | 6,240 | 80 | 7,170 |
Any other Asian background | <10 | 10 | 50 | 180 | 10 | 260 |
Caribbean | 230 | 1,420 | 5,180 | 11,330 | 920 | 19,090 |
African | 560 | 1,880 | 7,660 | 27,770 | 1,180 | 39,050 |
Any other Black / African / Caribbean background | <10 | 70 | 240 | 520 | 40 | 880 |
Arab | 120 | 330 | 1,280 | 3,920 | 300 | 5,950 |
Any other ethnic group | 10 | 10 | 20 | 50 | 10 | 90 |
Uncodeable | 2,490 | 10,190 | 36,070 | 85,190 | 7,540 | 141,470 |
Download this table Table 10: Number of children by highest level of attainment by ethnicity
.xls .csv
Ethnicity (NPD) | Highest level of educational attainment | |||||
---|---|---|---|---|---|---|
Below Level 1 | Level 1 | Level 2 | Level 3 | Missing | Total | |
British | 15,550 | 105,270 | 391,670 | 795,650 | 64,610 | 1,372,740 |
Irish | 80 | 360 | 1,220 | 4,200 | 230 | 6,080 |
Gypsy/Roma | 220 | 240 | 460 | 150 | 390 | 1,450 |
Traveller of Irish Heritage | 80 | 50 | 90 | 50 | 80 | 340 |
Any other white background | 910 | 3,430 | 12,790 | 32,600 | 2,520 | 52,260 |
White and Black Caribbean | 250 | 1,780 | 5,920 | 10,300 | 1,380 | 19,620 |
Black - African | 70 | 360 | 1,350 | 3,810 | 260 | 5,840 |
White and Asian | 140 | 670 | 2,530 | 8,960 | 390 | 12,700 |
Any other mixed background | 290 | 1,240 | 4,850 | 13,710 | 840 | 20,930 |
Pakistani | 710 | 3,610 | 12,170 | 30,800 | 2,060 | 49,350 |
Indian | 300 | 1,250 | 6,400 | 34,100 | 660 | 42,710 |
Bangladeshi | 220 | 1,450 | 4,780 | 14,110 | 730 | 21,290 |
Chinese | 40 | 110 | 660 | 5,910 | 70 | 6,780 |
Any other Asian Background | 300 | 940 | 4,000 | 15,710 | 670 | 21,620 |
Caribbean | 270 | 1,640 | 5,880 | 12,420 | 1,080 | 21,300 |
African | 600 | 2,070 | 8,410 | 29,010 | 1,410 | 41,490 |
Any other Black Background | 140 | 470 | 1,920 | 4,730 | 350 | 7,600 |
Any other Ethnic Group | 300 | 1,110 | 4,430 | 13,020 | 900 | 19,760 |
Refused | 120 | 730 | 2,610 | 6,550 | 410 | 10,420 |
Information not obtained | 250 | 620 | 2,130 | 4,930 | 580 | 8,500 |
Download this table Table 11: Number of children by highest level of attainment by ethnicity of child (Ethnicity taken from NPD)
.xls .csvIntersectionality
Male | Disability = No | ||||||||
---|---|---|---|---|---|---|---|---|---|
No religion | Christian | Buddhist | Hindu | Jewish | Muslim | Sikh | Other | Total | |
White British | 213,640 | 379,680 | 430 | 70 | 1,690 | 1,170 | 40 | 870 | 597,570 |
Mixed/Multiple Ethnic Groups | 8,040 | 11,450 | 120 | 160 | 40 | 1,480 | 100 | 80 | 21,460 |
Asian/Asian British | 2,660 | 2,210 | 390 | 7,640 | 20 | 33,910 | 5,850 | 490 | 53,160 |
Black/African/Caribbean/ Black British | 1,180 | 19,040 | <10 | 10 | <10 | 4,850 | <10 | 50 | 25,140 |
Other Ethnic Group | 50 | 130 | <10 | <10 | <10 | 2,570 | <10 | <10 | 2,750 |
Disability = Yes | |||||||||
White British | 14,950 | 23,830 | 40 | <10 | 100 | 70 | <10 | 120 | 39,110 |
Mixed/Multiple Ethnic Groups | 540 | 830 | <10 | <10 | <10 | 100 | <10 | <10 | 1,460 |
Asian/Asian British | 70 | 60 | 20 | 240 | <10 | 1,800 | 180 | 10 | 2,370 |
Black/African/Caribbean/ Black British | 80 | 880 | <10 | <10 | <10 | 190 | <10 | <10 | 1,140 |
Other Ethnic Group | <10 | <10 | <10 | <10 | <10 | 130 | <10 | <10 | 130 |
Download this table Table 12: Number of male children by ethnicity, religion and disability of the child
.xls .csv
Female | Disability = No | ||||||||
---|---|---|---|---|---|---|---|---|---|
No religion | Christian | Buddhist | Hindu | Jewish | Muslim | Sikh | Other | Total | |
White British | 198,100 | 387,560 | 550 | 40 | 1,970 | 1,090 | 20 | 1,150 | 590,480 |
Mixed/Multiple Ethnic Groups | 7,840 | 11,990 | 150 | 130 | 40 | 1,370 | 80 | 80 | 21,670 |
Asian/Asian British | 2,620 | 2,320 | 390 | 7,400 | 20 | 34,160 | 5,530 | 450 | 52,880 |
Black/African/Caribbean/ Black British | 1,060 | 20,560 | <10 | 20 | <10 | 4,710 | <10 | 30 | 26,380 |
Other Ethnic Group | 40 | 100 | <10 | <10 | <10 | 2,440 | <10 | <10 | 2,580 |
Disability = Yes | |||||||||
White British | 9,260 | 17,010 | 50 | <10 | 80 | 40 | <10 | 110 | 26,560 |
Mixed/Multiple Ethnic Groups | 370 | 550 | <10 | <10 | <10 | 60 | <10 | <10 | 970 |
Asian/Asian British | 40 | 50 | <10 | 160 | <10 | 1,500 | 150 | 10 | 1,920 |
Black/African/Caribbean/ Black British | 50 | 680 | <10 | <10 | <10 | 150 | <10 | <10 | 880 |
Other Ethnic Group | <10 | <10 | <10 | <10 | <10 | 100 | <10 | <10 | 100 |
Download this table Table 13: Number of female children by ethnicity, religion and disability of the child
.xls .csvFamilial characteristics and educational attainment
The relationship information used to derive household structure for the familial analysis comes from the 2011 Census. Each census form collected information for a maximum of six people, with households of more than six people having to request supplementary form(s) to provide information on remaining household members. Relationship information captured on supplementary forms links only to the first member of the household, meaning that the family structure of households with more than six members cannot be derived completely, and so these households have been excluded from this analysis.
The scope of this analysis included exploring the differential impact of the sex of the parent and their characteristics on the outcomes of the child. The category “mother” was assigned to all female parents and “father” to all male parents. While deriving whether a family member was the child of interest’s mother or father, there were cases where parents had “invalid” recorded as their sex. As such, it was not possible in these cases to assign a category of “mother” or “father” and so these cases have been excluded from the parent analysis in Tables 20 to 27.
For households with multiple mothers or fathers, the parent analysis includes breakdowns for each mother and father, where Mother 1 is the first recorded mother within the household (based on “Person number” from the Census), Mother 2 is the second recorded mother, and so on.
It should be noted that there were a small number of households containing three or more parents. Because of the low number of such households, these have been removed from the analysis.
There were also a number of other dataset limitations that resulted in further decreases in the base population for this analysis. Identification issues that arose in household members post-linkage meant that a complete household structure could not be derived for some households, with these households being dropped before analysis began. These identification issues also meant that in some cases it was not possible to join relationship information with the characteristics of household members. Such cases have been excluded from the corresponding analysis.
No-parent households could not be directly identified for this analysis, instead the following figures refer to cases where the mother and father identities are missing. This could be where the parent’s sex was listed as “invalid” and was therefore excluded from the analysis, or where further identification issues arose.
Summary of exclusions:
households of more than six members
parents with “invalid” sex
where complete household structures could not be derived
where joining relationship information and characteristics of household member was not possible
cases where both mother and father identities were missing
Household characteristics
Household type | Highest level of educational attainment | |||||
---|---|---|---|---|---|---|
Below Level 1 | Level 1 | Level 2 | Level 3 | Missing | Total | |
Lone parent family - Male parent | 720 | 4,800 | 14,060 | 19,550 | 3,500 | 42,630 |
Lone parent family - Female parent | 6,990 | 40,810 | 131,310 | 193,550 | 30,450 | 403,100 |
Couple family - Married - No children | 190 | 830 | 2,340 | 4,090 | 640 | 8,080 |
Couple family - Married - Non step-family (all children belong to both members of the couple) | 7,740 | 44,990 | 200,630 | 590,590 | 23,280 | 867,220 |
Couple family - Married - Step-family | 1,380 | 12,570 | 44,010 | 66,440 | 6,640 | 131,030 |
Couple family - Same-sex civil partnership - No children | <10 | <10 | 10 | 10 | <10 | 20 |
Couple family - Same-sex civil partnership -Non step-family (all children belong to both members of the couple) | <10 | 20 | 40 | 70 | 10 | 140 |
Couple family - Same-sex civil partnership - Step-family | <10 | 50 | 200 | 280 | 30 | 560 |
Couple family - Cohabiting - No children | 120 | 830 | 2,730 | 4,150 | 510 | 8,320 |
Couple family - Cohabiting - Non step-family (all children belong to both members of the couple) | 1,270 | 9,770 | 34,430 | 54,340 | 6,090 | 105,900 |
Couple family - Cohabiting - Step-family | 840 | 7,060 | 22,470 | 26,020 | 4,330 | 60,720 |
No code required/missing | 1,660 | 6,120 | 23,590 | 84,570 | 4,480 | 120,410 |
Download this table Table 14: Number of children by highest educational attainment by household type
.xls .csv
Household type of unemployed households | Highest level of educational attainment | |||||
---|---|---|---|---|---|---|
Below Level 1 | Level 1 | Level 2 | Level 3 | Missing | Total | |
Lone parent family - Male parent | <10 | 70 | 220 | 450 | 30 | 760 |
Lone parent family - Female parent | 20 | 400 | 1,840 | 4,650 | 150 | 7,060 |
Couple family - Married - No children | <10 | <10 | 20 | 60 | <10 | 80 |
Couple family - Married - Non step-family (all children belong to both members of the couple) | 10 | 350 | 2,610 | 11,480 | 120 | 14,560 |
Couple family - Married - Step-family | <10 | 130 | 730 | 1,720 | 40 | 2,620 |
Couple family - Same-sex civil partnership - No children | <10 | <10 | <10 | <10 | <10 | <10 |
Couple family - Same-sex civil partnership - Non step-family (all children belong to both members of the couple) | <10 | <10 | <10 | <10 | <10 | <10 |
Couple family - Same-sex civil partnership - Step-family | <10 | <10 | <10 | <10 | <10 | <10 |
Couple family - Cohabiting - No children | <10 | 10 | 50 | 100 | <10 | 160 |
Couple family - Cohabiting - Non step-family (all children belong to both members of the couple) | <10 | 80 | 420 | 1,100 | 30 | 1,630 |
Couple family - Cohabiting - Step-family | <10 | 70 | 390 | 610 | 30 | 1,100 |
No code required/ Missing | <10 | 50 | 380 | 2,060 | 30 | 2,510 |
Download this table Table 15: Number of children by highest educational attainment by household composition of unemployed households
.xls .csv
Number of members in the household who have a disability | Highest level of educational attainment | |||||
---|---|---|---|---|---|---|
Below Level 1 | Level 1 | Level 2 | Level 3 | Missing | Total | |
None | 12,450 | 85,540 | 339,270 | 812,100 | 48,120 | 1,297,480 |
One | 4,830 | 25,080 | 84,170 | 147,310 | 18,450 | 279,830 |
Two | 1,360 | 5,700 | 17,880 | 26,880 | 4,760 | 56,580 |
Three | 270 | 900 | 2,540 | 3,350 | 880 | 7,940 |
Four | 60 | 150 | 390 | 420 | 140 | 1,160 |
Five | <10 | 20 | 40 | 70 | 20 | 160 |
Missing | 1,940 | 10,460 | 31,510 | 53,510 | 7,570 | 104,990 |
Download this table Table 16: Number of children by highest educational attainment by number of members in the household who have a disability
.xls .csv
Siblings | Highest level of educational attainment | |||||
---|---|---|---|---|---|---|
Below Level 1 | Level 1 | Level 2 | Level 3 | Missing | Total | |
No Siblings | 6,040 | 33,050 | 112,920 | 205,660 | 22,830 | 380,490 |
One Sibling | 7,480 | 48,570 | 201,780 | 505,100 | 27,380 | 790,300 |
Two Siblings | 4,780 | 30,470 | 112,340 | 246,710 | 18,570 | 412,860 |
Three Siblings | 2,240 | 14,020 | 44,520 | 81,210 | 9,550 | 151,520 |
Four Siblings | 400 | 1,720 | 4,220 | 4,950 | 1,620 | 12,910 |
Five Siblings | <10 | <10 | 20 | 20 | <10 | 40 |
Download this table Table 17: Number of children by number of siblings and highest educational attainment
.xls .csv
Number of siblings aged under 16 years | Highest level of attainment | ||||||
---|---|---|---|---|---|---|---|
Below Level 1 | One | Two | Three | Four | Five | Total | |
One | 3,760 | 1,250 | 5,140 | 3,660 | 2,770 | 1,810 | 107,280 |
Two | 3,680 | 1,930 | 4,560 | 1,670 | 930 | <10 | 10,110 |
Three | 1,950 | 740 | 1,180 | 450 | <10 | <10 | 2,950 |
Four | 360 | 260 | 280 | <10 | <10 | <10 | 750 |
Five | <10 | 100 | <10 | <10 | <10 | <10 | 230 |
Missing | 11,160 | <10 | <10 | <10 | <10 | <10 | 50 |
Download this table Table 18: Number of children by highest educational attainment and number of siblings aged 16 and under (Household relationships taken from Census 2011)
.xls .csv
Number of siblings | Number of other relations in the household | ||||||
---|---|---|---|---|---|---|---|
No other relations | One other relation | Two other relations | Three other relations | Four other relations | Five other relations | Total | |
No siblings | 92,640 | 1,250 | 5,140 | 3,660 | 2,770 | 1,810 | 107,280 |
One sibling | 1,020 | 1,930 | 4,560 | 1,670 | 930 | <10 | 10,110 |
Two siblings | 580 | 740 | 1,180 | 450 | <10 | <10 | 2,950 |
Three siblings | 210 | 260 | 280 | <10 | <10 | <10 | 750 |
Four siblings | 130 | 100 | <10 | <10 | <10 | <10 | 230 |
Five siblings | 50 | <10 | <10 | <10 | <10 | <10 | 50 |
Download this table Table 19: Number of children by number of siblings and number of other relations in households where there are no parents and no grandparents recorded
.xls .csvCharacteristics of mother
Carer status of Mother 1 | Highest level of educational attainment | |||||
---|---|---|---|---|---|---|
Below Level 1 | Level 1 | Level 2 | Level 3 | Missing | Total | |
No | 6,530 | 84,710 | 334,270 | 771,170 | 43,890 | 1,240,570 |
Yes, 1 to 19 hours a week | 850 | 8,950 | 41,180 | 119,250 | 4,700 | 174,930 |
Yes, 20 to 49 hours a week | 1,190 | 3,720 | 12,070 | 19,490 | 3,560 | 40,030 |
Yes, 50 hours and over a week | 8,290 | 8,470 | 23,260 | 31,520 | 11,010 | 82,550 |
Failed multiple ticking rules | 30 | 60 | 160 | 240 | 60 | 550 |
Missing | 3,940 | 21,480 | 63,310 | 99,030 | 16,410 | 204,170 |
Download this table Table 20: Number of children by highest educational attainment by carer status of Mother 1
.xls .csv
Carer status of Mother 2 | Highest level of educational attainment | |||||
---|---|---|---|---|---|---|
Below Level 1 | Level 1 | Level 2 | Level 3 | Missing | Total | |
No | 30 | 240 | 770 | 1,440 | 120 | 2,600 |
Yes, 1 to 19 hours a week | <10 | 20 | 70 | 180 | 20 | 290 |
Yes, 20 to 49 hours a week | <10 | 10 | 30 | 40 | 10 | 100 |
Yes, 50 hours and over a week | 20 | 30 | 60 | 70 | 30 | 200 |
Failed multiple ticking rules/missing | 20,780 | 127,080 | 473,330 | 1,038,970 | 79,430 | 1,739,590 |
Download this table Table 21: Number of children by highest educational attainment by carer status of Mother 2
.xls .csv
NSSEC of Mother 1 | Highest level of educational attainment | |||||
---|---|---|---|---|---|---|
Below Level 1 | Level 1 | Level 2 | Level 3 | Missing | Total | |
Higher managerial and professional occupations | 550 | 2,420 | 13,700 | 72,330 | 1,160 | 90,160 |
Lower managerial and professional occupations | 740 | 6,580 | 29,100 | 72,580 | 3,120 | 112,110 |
Intermediate occupations | 2,390 | 16,920 | 80,820 | 226,190 | 7,710 | 334,030 |
Small employers and own account workers | 830 | 6,110 | 26,830 | 64,520 | 2,860 | 101,140 |
Lower supervisory and technical occupations | 570 | 5,300 | 18,900 | 29,530 | 2,880 | 57,180 |
Semi-routine occupations | 3,570 | 26,610 | 94,240 | 142,760 | 15,870 | 283,040 |
Routine occupations | 2,380 | 15,060 | 48,190 | 59,960 | 10,370 | 135,960 |
Missing | 9,810 | 48,380 | 162,480 | 372,840 | 35,660 | 629,170 |
Download this table Table 22: Number of children by highest educational attainment by National Statistics Socio-Economic Classification (NSSEC) of Mother 1
.xls .csv
NSSEC of Mother 2 | Highest level of educational attainment | |||||
---|---|---|---|---|---|---|
Below Level 1 | Level 1 | Level 2 | Level 3 | Missing | Total | |
Higher managerial and professional occupations | <10 | <10 | 50 | 190 | <10 | 250 |
Lower managerial and professional occupations | <10 | 20 | 60 | 170 | 10 | 270 |
Intermediate occupations | <10 | 30 | 90 | 180 | 10 | 300 |
Small employers and own account workers | <10 | 30 | 120 | 180 | 20 | 350 |
Lower supervisory and technical occupations | <10 | 30 | 60 | 120 | 20 | 220 |
Semi-routine occupations | 10 | 60 | 150 | 210 | 30 | 460 |
Routine occupations | <10 | 50 | 130 | 140 | 20 | 350 |
Missing | 10 | 127,150 | 473,590 | 1,039,510 | 79,500 | 1,740,540 |
Download this table Table 23: Number of children by highest educational attainment by National Statistics Socio-Economic Classification (NSSEC) of Mother 2
.xls .csvCharacteristics of father
Carer status of Father 1 | Highest level of educational attainment | |||||
---|---|---|---|---|---|---|
Below Level 1 | Level 1 | Level 2 | Level 3 | Missing | Total | |
No | 4,790 | 61,150 | 256,540 | 663,460 | 29,080 | 1,015,020 |
Yes, 1 to 19 hours a week | 1,250 | 4,450 | 21,780 | 72,190 | 2,750 | 102,420 |
Yes, 20 to 49 hours a week | 1,230 | 1,740 | 5,770 | 10,090 | 1,760 | 20,590 |
Yes, 50 hours and over a week | 3,540 | 3,280 | 9,300 | 13,650 | 3,930 | 33,710 |
Failed multiple ticking rules | 10 | 30 | 70 | 140 | 20 | 270 |
Missing | 10,010 | 56,730 | 180,800 | 281,160 | 42,080 | 570,790 |
Total | 20,830 | 127,380 | 474,260 | 1,040,700 | 79,620 | 1,742,790 |
Download this table Table 24: Number of children by highest educational attainment by carer status of Father 1
.xls .csv
Carer status of Father 2 | Highest level of educational attainment | |||||
---|---|---|---|---|---|---|
Below Level 1 | Level 1 | Level 2 | Level 3 | Missing | Total | |
No | <10 | 80 | 280 | 650 | 40 | 1060 |
Yes, 1 to 19 hours a week | <10 | <10 | 30 | 90 | <10 | 120 |
Yes, 20 to 49 hours a week | <10 | <10 | 20 | 20 | <10 | 40 |
Yes, 50 hours and over a week | <10 | <10 | 20 | 20 | <10 | 40 |
Failed multiple ticking rules | <10 | <10 | <10 | <10 | <10 | <10 |
Download this table Table 25: Number of children by highest educational attainment by carer status of Father 2
.xls .csv
NSSEC of Father 1 | Highest level of educational attainment | |||||
---|---|---|---|---|---|---|
Below Level 1 | Level 1 | Level 2 | Level 3 | Missing | Total | |
Higher managerial and professional occupations | 1,380 | 4,860 | 28,490 | 161,960 | 2,250 | 198,930 |
Lower managerial and professional occupations | 910 | 5,820 | 29,020 | 96,570 | 2,450 | 134,770 |
Intermediate occupations | 500 | 3,150 | 15,210 | 45,130 | 1,490 | 65,460 |
Small employers and own account workers | 1,760 | 14,210 | 59,460 | 129,560 | 6,480 | 211,460 |
Lower supervisory and technical occupations | 1,110 | 9,240 | 38,000 | 70,470 | 4,340 | 123,150 |
Semi-routine occupations | 1,220 | 8,920 | 32,740 | 57,670 | 5,120 | 105,680 |
Routine occupations | 1,690 | 14,120 | 48,190 | 66,020 | 8,420 | 138,440 |
Missing | 12,290 | 67,070 | 223,140 | 413,320 | 49,080 | 764,900 |
Download this table Table 26: Number of children by highest educational attainment by National Statistics Socio-Economic Classification (NSSEC) of Father 1
.xls .csv
NSSEC of Father 2 | Highest level of educational attainment | |||||
---|---|---|---|---|---|---|
Below Level 1 | Level 1 | Level 2 | Level 3 | Missing | Total | |
Higher managerial and professional occupations | <10 | <10 | 10 | 50 | <10 | 63 |
Lower managerial and professional occupations | <10 | <10 | 30 | 50 | <10 | 80 |
Intermediate occupations | <10 | 10 | 50 | 140 | <10 | 200 |
Small employers and own account workers | <10 | <10 | 40 | 80 | <10 | 120 |
Lower supervisory and technical occupations | <10 | <10 | 20 | 40 | <10 | 60 |
Semi-routine occupations | <10 | 20 | 50 | 90 | <10 | 160 |
Routine occupations | <10 | 20 | 50 | 70 | 20 | 140 |
Missing | 20,830 | 127,310 | 474,020 | 1,040,180 | 79,580 | 1,741,920 |
Download this table Table 27: Number of children by highest educational attainment by National Statistics Socio-Economic Classification (NSSEC) of Father 2
.xls .csvCharacteristics of parents by educational attainment
Number of parents with a disability | Highest level of educational attainment | |||||
---|---|---|---|---|---|---|
No qualifications | Level 1 | Level 2 | Level 3 | Missing | Total | |
No Parents with a disability | 16,170 | 102,550 | 391,030 | 899,760 | 61,130 | 1,470,640 |
One Parent with a disability | 4,110 | 22,220 | 74,580 | 127,480 | 16,390 | 244,770 |
Two Parents with a disability | 650 | 3,070 | 10,180 | 16,400 | 2,420 | 32,720 |
Download this table Table 28: Highest educational attainment of child by number of parents with a disability
.xls .csv
Disability status of parent in a lone male parent household | Highest level of educational attainment | |||||
---|---|---|---|---|---|---|
No Qualifications | Level 1 | Level 2 | Level 3 | Missing | Total | |
Parent does not have a disability | 550 | 3,820 | 11,450 | 16,840 | 2,570 | 35,230 |
Parent has a disability | 180 | 980 | 2,610 | 2,700 | 9,20 | 7,390 |
Download this table Table 29: Highest educational attainment of child by whether parent of a lone male parent household has a disability
.xls .csv
Disability status of parent in a lone female parent household | Highest level of educational attainment | |||||
---|---|---|---|---|---|---|
Below Level 1 | Level 1 | Level 2 | Level 3 | Missing | Total | |
Parent does not have a disability | 5,360 | 33,180 | 109,090 | 167,190 | 23,610 | 338,430 |
Parent has a disability | 1,630 | 7,630 | 22,200 | 26,340 | 6,830 | 64,620 |
Download this table Table 30: Highest educational attainment of child by whether parent of a lone female parent household has a disability
.xls .csv
Country of birth of parents | Highest level of educational attainment | |||||
---|---|---|---|---|---|---|
Below Level 1 | Level 1 | Level 2 | Level 3 | Missing | Total | |
UK-born | 14,380 | 98,740 | 374,300 | 771,580 | 59,520 | 1,318,510 |
One parent Non-UK born | 2,110 | 9,300 | 36,680 | 117,550 | 5,570 | 171,210 |
Two parents Non-UK born | 1,500 | 5,060 | 21,920 | 88,420 | 3,210 | 120,120 |
Missing | 2,930 | 14,750 | 42,890 | 66,080 | 11,640 | 138,290 |
Download this table Table 31: Highest educational attainment of child by country of birth of parents
.xls .csv
Main language of parents | Highest level of educational attainment | |||||
---|---|---|---|---|---|---|
Below Level 1 | Level 1 | Level 2 | Level 3 | Missing | Total | |
English | 11,760 | 75,300 | 300,220 | 728,430 | 42,510 | 1,158,210 |
One parent non-English | 390 | 1,610 | 6,540 | 24,140 | 880 | 33,560 |
Two parent's non-English | 970 | 3,700 | 15,290 | 52,170 | 2,400 | 74,520 |
Missing | 7,810 | 47,240 | 153,740 | 238,900 | 34,140 | 481,840 |
Download this table Table 32: Highest educational attainment of child by main language of parents
.xls .csv
Parents English language proficiency | Highest level of educational attainment | |||||
---|---|---|---|---|---|---|
Below Level 1 | Level 1 | Level 2 | Level 3 | Missing | Total | |
Both well | 16,180 | 102,580 | 383,120 | 831,120 | 63,250 | 1,396,240 |
One parent not well | 330 | 1,420 | 5,520 | 14,660 | 920 | 22,850 |
Two parents not well | 210 | 900 | 2,990 | 6,430 | 730 | 11,260 |
Missing | 4,210 | 22,930 | 84,160 | 191,440 | 15,050 | 317,790 |
Download this table Table 33: Highest educational attainment of child by parents’ English language proficiency
.xls .csvVulnerable groups and educational attainment
There are a number of characteristics used by the Office of the Children’s Commissioner for England (CCO) to identify vulnerable children. The characteristics of vulnerable children that are captured within the Proof of Concept (PoC) dataset are children from minority ethnic backgrounds, young carers, and lone-parent families.
Not Young Carers | Ethnicity | |||||
---|---|---|---|---|---|---|
White British | Mixed/ Multiple Ethnicity | Asian/ Asian British | Black/ African/ Caribbean | Other | Total | |
Lone parent family - Male parent | 32,150 | 1,400 | 1,520 | 1,400 | 120 | 36,600 |
Lone parent family - Female parent | 283,560 | 18,890 | 14,730 | 24,300 | 1,190 | 342,670 |
Couple family - Married - No children | 4,460 | 200 | 1,420 | 180 | 20 | 6,280 |
Couple family - Married - Non step-family (all children belong to both members of the couple) | 642,630 | 15,340 | 79,180 | 17,300 | 3,750 | 758,190 |
Couple family - Married - Step-family | 107,050 | 3,200 | 2,970 | 3,040 | 190 | 116,450 |
Couple family - Same-sex civil partnership - No children | 20 | <10 | <10 | <10 | <10 | 20 |
Couple family - Same-sex civil partnership - Non step-family (all children belong to both members of the couple) | 110 | <10 | <10 | <10 | <10 | 110 |
Couple family - Same-sex civil partnership - Step-family | 490 | 10 | <10 | <10 | <10 | 500 |
Couple family - Cohabiting - No children | 6,560 | 230 | 210 | 170 | 10 | 7,180 |
Couple family - Cohabiting - Non step-family (all children belong to both members of the couple) | 87,760 | 3,360 | 1,330 | 1,940 | 80 | 94,460 |
Couple family - Cohabiting - Step-family | 51,330 | 1,700 | 390 | 870 | 20 | 54,310 |
No code required/Missing | 80,130 | 3,190 | 9,240 | 5,770 | 360 | 98,690 |
Download this table Table 34: Number of children by household type, ethnicity and carer status
.xls .csv
Young carers | Ethnicity | |||||
---|---|---|---|---|---|---|
White British | Mixed/ Multiple Ethnicity | Asian/ Asian British | Black/ African/ Caribbean | Other | Total | |
Lone parent family - Male parent | 1,360 | 60 | 100 | 50 | <10 | 1,560 |
Lone parent family - Female parent | 11,600 | 890 | 800 | 850 | 60 | 14,210 |
Couple family - Married - No children | 170 | <10 | 80 | <10 | <10 | 250 |
Couple family - Married - Non step-family (all children belong to both members of the couple) | 18,800 | 480 | 2,940 | 430 | 90 | 22,720 |
Couple family - Married - Step-family | 3,070 | 90 | 110 | 60 | <10 | 3,330 |
Couple family - Same-sex civil partnership - No children | <10 | <10 | <10 | <10 | <10 | . |
Couple family - Same-sex civil partnership - Non step-family (all children belong to both members of the couple) | <10 | <10 | <10 | <10 | <10 | . |
Couple family - Same-sex civil partnership - Step-family | 30 | <10 | <10 | <10 | <10 | 30 |
Couple family - Cohabiting - No children | 150 | <10 | 10 | <10 | <10 | 160 |
Couple family - Cohabiting - Non step-family (all children belong to both members of the couple) | 2,360 | 100 | 60 | 50 | <10 | 2,570 |
Couple family - Cohabiting - Step-family | 1,310 | 50 | 10 | 20 | <10 | 1,390 |
No code required/Missing | 2,470 | 120 | 500 | 150 | <10 | 3,240 |
Download this table Table 35: Number of children by household type, ethnicity and carer status
.xls .csvGeography and educational attainment
Mobility indicator (NPD) | Highest Level of Attainment | |||||
---|---|---|---|---|---|---|
Below Level 1 | Level 1 | Level 2 | Level 3 | Missing | Total | |
Postcode unchanged | 16,900 | 112,850 | 432,030 | 980,020 | 65,530 | 1,607,340 |
Postcode change between spring School Census | 2,100 | 12,230 | 36,030 | 50,750 | 9,440 | 110,550 |
Incomplete address in previous spring school Census | 780 | 1,130 | 3,930 | 7,880 | 1,610 | 15,330 |
Incomplete address in current spring school Census | 80 | 340 | 1,060 | 1,650 | 250 | 3,370 |
Missing | 980 | 830 | 1,210 | 400 | 2,790 | 6,200 |
Download this table Table 36: Highest educational attainment of child by change in address from previous Spring Census (Mobility indicator taken from NPD)
.xls .csv
Urban and rural geographies | Below Level 1 | Level 1 | Level 2 | Level 3 | Missing | Total |
---|---|---|---|---|---|---|
Urban: Major Conurbation | 7,370 | 37,340 | 151,520 | 353,820 | 28,370 | 578,430 |
Urban: Minor Conurbation | 790 | 5,130 | 18,240 | 32,050 | 3,820 | 60,030 |
Urban: City and Town | 8,860 | 59,900 | 210,680 | 423,520 | 35,370 | 738,320 |
Urban: City and Town in a Sparse Setting | 50 | 200 | 1,040 | 1,410 | 140 | 2,830 |
Rural Town and Fringe | 1,470 | 10,360 | 38,350 | 89,290 | 4,980 | 144,440 |
Rural Town and Fringe in a Sparse Setting | 70 | 530 | 1,780 | 3,030 | 210 | 5,630 |
Rural Village | 800 | 5,250 | 19,880 | 56,240 | 2,240 | 84,400 |
Rural Village in Sparse Setting | 50 | 380 | 1,470 | 3,150 | 170 | 5,210 |
Rural Hamlet and isolated dwellings | 690 | 4,160 | 15,980 | 46,420 | 1,760 | 69,000 |
Rural Hamlet and isolated dwellings in a Sparse Setting | 40 | 360 | 1,460 | 3,370 | 120 | 5,360 |
Missing | 650 | 3,780 | 13,870 | 28,410 | 2,430 | 49,150 |
Download this table Table 37: Highest educational attainment of child by urban and rural geographies
.xls .csv7. Quality and methodology
Dimensions of quality
To ensure a broad understanding of our work and quality, within this work, we adhere to the Code of Practice for Official Statistics (PDF, 5.77KB) and use the European Statistical System’s Dimensions of Quality to inform users about the quality of the data.
Data preparation of the All Education Dataset for England (AEDE)
Overview
To build the dataset, we have taken a series of preparatory steps which included pre-processing of data and hashing of data (described under data preparation). Before describing the linkage methodology in more detail, Figure 3 provides an overview of the linkage work and the datasets referred to in this article.
Figure 3: Flowchart of each dataset referred to in this article, and used in the feasibility AEDE-Census linkage
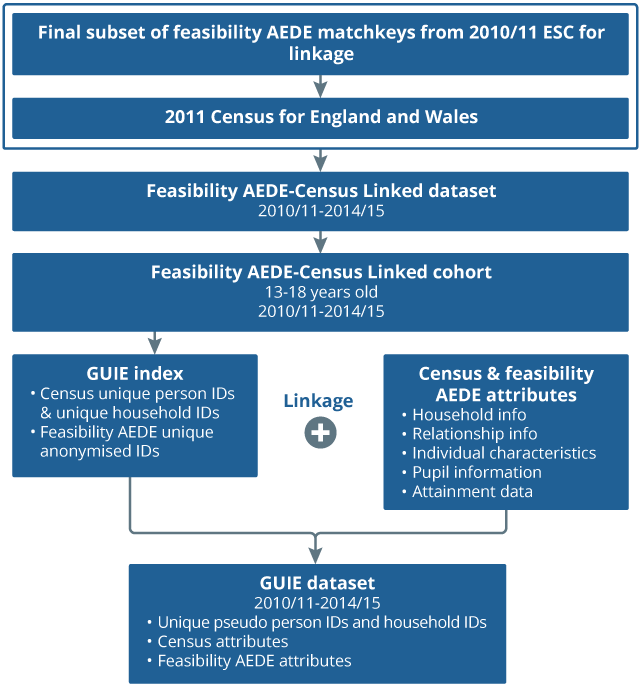
Source: Office for National Statistics
Download this image Figure 3: Flowchart of each dataset referred to in this article, and used in the feasibility AEDE-Census linkage
.png (46.5 kB)Data preparation The pre-processing of data included geo-referencing, variable standardisation and matchkey creation. Geo-referencing involves referencing data to a specific and fixed point, using a geographic classification and a grid of reference. Through variable standardisation, all variables are placed on the same scale to allow for comparisons. For example, if an individual’s forename is recorded as Anne-Marie on one dataset, but as Annemarie on the other, the standardisation removes non-alphabet characters and capitalises them, so the name will appear as ANNEMARIE on both datasets and this forename will link post encryption. Standardisation of variables includes cleaning linkage variables on all data sources to improve linkage rates. Additional processing is completed on the linkage variables to build matchkeys.
To protect confidentiality, all personal data used to create the Growing Up in England (GUIE) linked dataset such as names and dates of birth were pseudonymised (made non-identifiable) through a pseudonymisation process. Data pseudonymisation, or hashing, is a one-way, irreversible process where data are pseudonymised by transforming the raw, identifiable data into a unique string of letters and numbers. The nature of the hashing process means that only in cases where two records are identical, where names, dates of birth and addresses are recorded in precisely the same format, will an automatic match be possible on the hashed values. In cases where there are spelling errors or inconsistencies between two records relating to the same individual (for example the names Samantha and Sam or John and Jon), the hash values will not be identifiable as being similar.
Feasibility AEDE data processing
The feasibility AEDE consists of several files of data with information on attainment, pupil attributes and pseudonymised person identifiers all separated. To use the data for linkage, multiple steps were required to pull different aspects of an individual together. The process for building the feasibility AEDE subset for linkage is described next, and illustrated in Figure 4.
First, the feasibility AEDE attributes containing the source and academic year variables were linked to the AEDE index on the Pupil Matching Reference (PMR) number. The PMR gives each pupil an pseudonymised identifier, which is unique to them and allows matching across datasets without giving away their identity. The purpose of this step was to get the Unique ID from the AEDE index onto the attributes which is needed to link to the matchkey file.
Once the feasibility AEDE attributes had been linked to the AEDE index, the AEDE index was subset into the 2010/11 academic year where School Census was identified as the source dataset. Multiple entries of individual records have been removed; this process is known as de-duplication which was completed on the PMR and using a nodupkey procedure in SAS. The nodupkey procedure retains only the first instance of a record.
Having removed all the duplicated records, the final AEDE subset for linkage contained 2,250,655 individuals. These individuals were then linked to the original matchkey file on the PMR to extract the correct matchkey records for linkage.
The resulting AEDE matchkey file, created for linkage, contained over 161 million records covering the academic years 2000/01 to 2014/15. For the purposes of this AEDE-Census linkage, the file was subset into the School Census records for the 2010/11 academic year, because this was the year closest to 2011 Census. This is important because the information collected in this year is most likely to match that of the Census, in particular a person’s address details, and so increasing the number of records which will link.
Figure 4: Preparation of feasibility AEDE
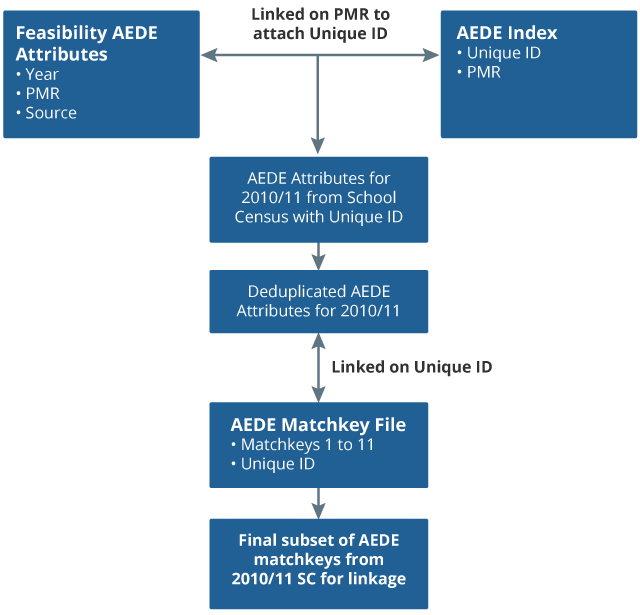
Source: Office for National Statistics
Download this image Figure 4: Preparation of feasibility AEDE
.png (30.1 kB)Creating matchkeys
Census data and the feasibility AEDE do not contain a single common identifier that could be used to easily link corresponding records from one dataset to the other, for example a unique number for each individual that is common to both datasets. Therefore, a series of matchkeys containing different combinations of pseudonymised person information, including name, date of birth, gender and postcode, was used to link the AEDE to the Census. For example, forename, surname, date of birth and postcode may be combined and for each member of the population would be expected to retain a high level of uniqueness. As previously mentioned, identifiable data were hashed and used to link records between datasets in the anonymous data research environment.
It is expected that administrative data and survey data will contain some level of error in the capture and quality of the information contained therein. These errors can prevent links being made where they should be (these missed matches are known as false negatives). To help reduce the likelihood of false negatives, nine matchkeys were created and used, some of which allow for small amounts of error within the identifier variables, for instance difference in name spellings or where gender may be missing. Each matchkey is designed to gradually eliminate some of the discrepancies that may otherwise prevent automated matching (Figure 4).
The matchkeys were run in order of strength, by which we mean how able the matchkey is to discern between truly different records. This matchkey ordering differs from the numbering of the matchkeys provided in Table 38. Matchkeys 1 to 11 allow only exact matches on all the selected variables. The standard available matchkeys, developed for use when matching data to the Census, and the information contained within them are shown in Table 38. For more detailed methodology on matchkeys and linking pseudonymised data, see Beyond 2011 data linkage methods (PDF, 319.9KB).
Matchkey | Matchkey identifiers |
---|---|
1 | FORENAME | SURNAME | DOB | GENDER | POSTCODE |
2 | FORENAME | SURNAME | DOB | GENDER |
3 | FORENAME INITIAL | SURNAME INITIAL | DOB | GENDER | POSTCODE DISTRICT |
4 | FORENAME INITIAL | DOB | GENDER | POSTCODE |
5 | SURNAME INITIAL | DOB | GENDER | POSTCODE |
6 | FORENAME | SURNAME | SEX | POSTCODE |
7 | FORENAME BIGRAM | SURNAME BIGRAM | DOB | SEX | POSTCODE AREA |
8 | FORENAME | SURNAME | YEAR OF BIRTH | GENDER | POSTCODE DISTRICT |
11 | FORENAME | SURNAME | DOB | POSTCODE |
Download this table Table 38: Matchkey detailed information used for linkage
.xls .csvDeterministic linking – Census to feasibility AEDE
Table 39 shows the linkage rates for each matchkey for all AEDE-Census records. Out of 2,250,655 records on the AEDE, 2,035,289 records (90%) linked to a corresponding Census record. Two-thirds (66.38%) of the linked AEDE records linked to the Census on the strongest matchkey (1) followed by 19.75%, which linked on matchkey 3.
To link the Census to AEDE, matchkeys from each data source are compared and if they agree, a match is established. Each matchkey is applied to the datasets in an order whereby the amount of error allowed between the datasets is increased gradually. In this way the best quality links are formed earlier. To avoid the possibility of creating false positive links (that is, matching records that should not be matched, for example, two different people) records are only linked on a matchkey if it is unique on both datasets. Where multiple records link on the same key, the link is disregarded and the records are passed on as a residual to the next pass. Matches made in the early stages are given priority over those made on later (weaker) links.
Census to AEDE | ||
---|---|---|
Matchkey | Count | % |
1 | 1,350,974 | 66.38 |
2 | 22,282 | 1.09 |
3 | 401,911 | 19.75 |
4 | 95,597 | 4.7 |
5 | 47,070 | 2.31 |
6 | 50,049 | 2.46 |
7 | 59,440 | 2.92 |
8 | 2,326 | 0.11 |
11 | 5,640 | 0.28 |
Total | 2,035,289 |
Download this table Table 39: Match rates by matchkey for the feasibility AEDE to Census 2011 linkage
.xls .csvOnce the AEDE-Census linkage was complete, a cohort of children aged 13 to 18 years on 31 August 2011 in the Census was extracted from this linked dataset to create the initial “index” of 1,920,091 records for the Growing Up in England (GUIE) build. These records were selected as they represent the analytical cohort. This index consists of the unique Census person identifiers and household identifiers, and the unique identifier numbers provided within the feasibility AEDE. The index was used as a base on which to build the Census and feasibility AEDE attributes tables. The unique IDs were replaced with pseudo IDs on all tables provided to researchers to protect the identity of individuals within the datasets.
The next step was to link in other household information collected in the Census, and pupil attributes and attainment from the feasibility AEDE, to the cohort to produce the Census and feasibility AEDE attributes tables for analysis. Figure 5 shows which tables of data were linked and produced.
Figure 5: Tables of data used for initial linkage and final tables for analysis
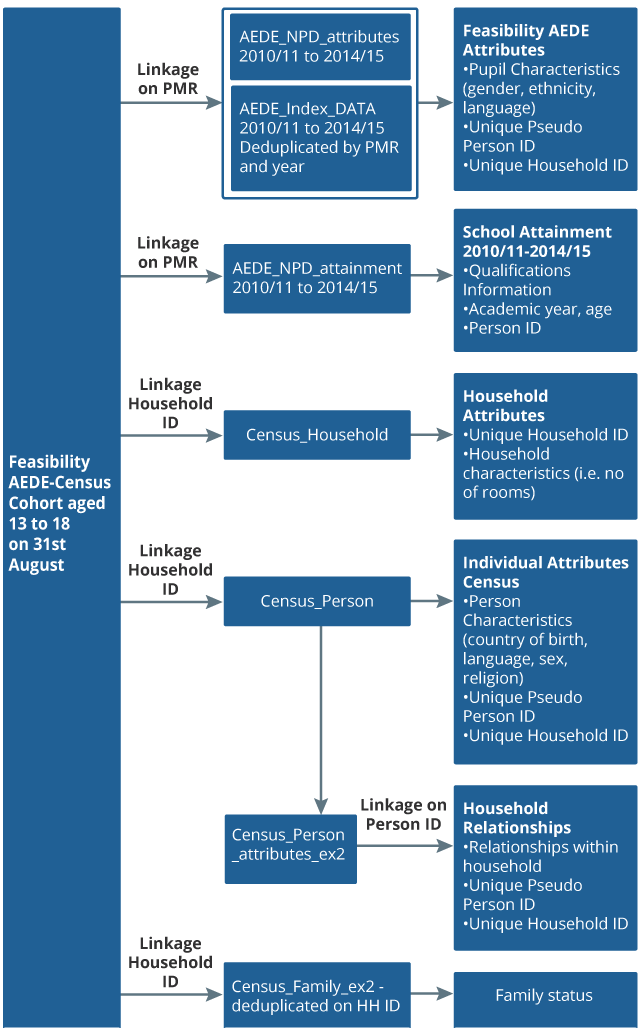
Source: Office for National Statistics
Notes:
- The tables on the right-hand side reflect the final tables in GUIE. These are: Feasibility AEDE Attributes, School Attainment between 2010 to 2011 and 2014 to 2015, Household Attributes, Individual Attributes Census, Household Relationships, and Family status.
Download this image Figure 5: Tables of data used for initial linkage and final tables for analysis
.png (79.7 kB)8. Linkage results
Quality checks
On the creation of the AEDE attainment tables, an anomaly was discovered by which, of the 1,920,091 records in the AEDE-Census linked cohort, only 1,236,071 linked to a record from academic year 2010/11 attainment data. Further investigation revealed that a number of individuals in the AEDE which had linked to the Census were found to be present in AEDE attainment from academic year 2011/12 onwards but were not found to be recorded in the 2010/11 academic year. The reason for this is unclear and may be a quality issue in the AEDE or as a result of lag in the recording of data in the AEDE. This anomaly means that the number of attainment records increases through the years. For the purposes of consistency, only cohort records that linked to an attainment record in academic year 2010/11 were retained in the attainment records for each of the academic years.
To evaluate the quality of the linkage, a series of age distributions was created (Figure 6).
Figure 6: Comparison of age distributions in feasibility AEDE-Census linked and unlinked feasibility AEDE data
Source: Office for National Statistics
Download this chart Figure 6: Comparison of age distributions in feasibility AEDE-Census linked and unlinked feasibility AEDE data
Image .csv .xlsSample sizes: Feasibility AEDE index 2010/11 – 7,143,303
Feasibility AEDE subset for linkage – 2,250,655
Feasibility AEDE-Census Linked cohort – 2,035,212
Unlinked Feasibility AEDE – 215,366
The age distributions show that, aside from the AEDE index 2010/11, there is consistency between the feasibility AEDE subset for linkage, feasibility AEDE-Census linked cohort and the unlinked feasibility AEDE, although the proportion of 14-year-olds in the unlinked AEDE is slightly higher.
The lower numbers of 13-year-olds in the linkage outputs reflect the lower proportion of this age within the AEDE index dataset. However, with regards to the low numbers of 17- and 18-year-olds, although they are consistent within the outputs, it is unclear as to why they would be much lower than those in the AEDE index dataset and may need further investigation going forward. In conclusion, consistency across age distribution indicates that there was no age bias in the linkage.
Unlinked feasibility AEDE records
215,366 AEDE records did not link to a Census record. This is 10% of the total number of records extracted for the linkage. Where we have seen that there are no sizeable differences in the age distributions shown in Figure 6, this suggests that age was not the cause of these records not linking.
Boarders were of particular interest because they may be listed at a different address on feasibility AEDE to their usual address on the Census. Therefore, a check on the numbers of boarders within the unlinked feasibility AEDE was carried out to check for any linkage bias (Table 40). It can be seen that 212,449 records of a total of 215,366 unlinked records (98%) were not boarders. Therefore, this is not solely responsible for records not linking to the Census.
Boarder status | Count |
---|---|
Boarder, six nights or fewer a week | 197 |
Boarder, seven nights a week | 69 |
Boarder, nights per week not specified | 964 |
Not a boarder | 212,449 |
Missing | 1,687 |
Total | 215,366 |
Download this table Table 40: Boarder status in unlinked AEDE records
.xls .csvOne of the limitations of linking pseudonymised data is that it is difficult to identify where specific errors in the recording of variables affect the ability for the algorithm to make matches. Without being able to clerically review identifiable record-level data, it is therefore difficult to correct for error in the variables, as well as difficult to calculate false positive and false negative errors (that is, records that should have been matched but were missed and records that did match that should not have).
Back to table of contents9. Lessons learned and next steps
This report showed that a sufficiently high proportion of records linked between these datasets, and the majority of these were made on strong matchkeys, giving confidence that those links are correct; this demonstrates the feasibility of the linkage. It is recommended therefore that research under the Growing Up in England (GUIE) theme should continue and should consider linkage as an important part of that work.
In this specific case, it would be interesting to further investigate the linkage quality using clerical samples as well as to answer outstanding questions such as why 17- and 18-year olds appear differently in age distributions.
Where individuals in the feasibility AEDE attainment data were not found to be in the 2010/11 academic year but appeared in later years, the linked attainment tables were re-run to provide consistency. This involved linking the cohort to the 2010/11 academic year of attainment and only bringing those cohort members into the longitudinal file. This step can be incorporated into the methodology for the future GUIE build going forward into the new linkage environment.
These, together with the issues identified during the analysis stage, will help inform the future iterations of the GUIE dataset. This PoC dataset is a feasibility study on the potential of linked administrative data.
The next steps for the GUIE project include the re-creation of this linked dataset, called GUIE Wave 1, in a new linkage environment at the Office for National Statistics (ONS). This will allow for a better matching exercise, as the quality of matches can be reviewed when linkage is conducted. This opens the potential for creating additional matchkeys that can consider more and different types of error. The GUIE Wave 1 dataset will be made available to accredited researchers with approved projects to use via the Secure Research Service (SRS). The expected delivery of this dataset into the SRS is late 2020.
Back to table of contents10. Annex A: Glossary
Data linkage
The act of bringing two or more datasets from different sources together, creating associations between the data. Data linkage can provide new statistical insights not possible with information from a single source.
Data processing
Data processing is the method applied to convert data into a format that can be interpreted, analysed and used for a variety of purposes.
Data quality
An essential characteristic that determines the reliability of data for making decisions. High-quality data are complete, accurate, available and timely.
De-identified
De-identified data do not contain any personal identifiable information, such as name, address, postcodes etc. The identifiers are removed from the records before de-identified microdata is securely transferred to the Secure Research System (the secure environment where access is controlled).
Back to table of contents